Comparison of Binary Diagnostic Tests in a Paired Study Design.
DTComPair 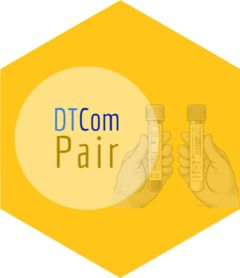
Comparison of the accuracy of two binary diagnostic tests in a “paired” study design, i.e. when each test is applied to each subject in the study.
Installation
CRAN
You can install the current stable version from CRAN with:
install.packages("DTComPair")
GitHub
You can install the current development version from GitHub with:
if (!require("remotes")) {install.packages("remotes")}
remotes::install_github("chstock/DTComPair")
Scope
Diagnostic accuracy measures that can be computed and compared are sensitivity, specificity, positive and negative predictive values, and positive and negative diagnostic likelihood ratios.
Getting Started
Determine the accuracy of one diagnostic test
library(DTComPair)
# Loading required package: PropCIs
data(Paired1) # Hypothetical study data
a1 <- tab.1test(d=d, y=y1, data=Paired1)
print(a1)
# Binary diagnostic test 'y1'
#
# Diseased Non-diseased Total
# Test pos. 397 84 481
# Test neg. 54 177 231
# Total 451 261 712
a2 <- acc.1test(a1)
print(a2)
# Diagnostic accuracy of test 'y1'
#
# (Estimates, standard errors and 95%-confidence intervals)
#
# Est. SE Lower CL Upper CL
# Sensitivity 0.8802661 0.01528718 0.8503038 0.9102284
# Specificity 0.6781609 0.02891782 0.6214830 0.7348388
# PPV 0.8253638 0.01731081 0.7914353 0.8592924
# NPV 0.7662338 0.02784617 0.7116563 0.8208113
#
# Est. SE (log) Lower CL Upper CL
# PDLR 2.7351124 0.0915147 2.2860079 3.2724472
# NDLR 0.1765568 0.1346088 0.1356142 0.2298601
Compare the accuracy of two diagnostic tests
Compute accuracy measures
b1 <- tab.paired(d=d, y1=y1, y2=y2, data=Paired1)
print(b1)
# Two binary diagnostic tests (paired design)
#
# Test1: 'y1'
# Test2: 'y2'
#
# Diseased:
# Test1 pos. Test1 neg. Total
# Test2 pos. 319 22 341
# Test2 neg. 78 32 110
# Total 397 54 451
#
# Non-diseased:
# Test1 pos. Test1 neg. Total
# Test2 pos. 31 22 53
# Test2 neg. 53 155 208
# Total 84 177 261
b2 <- acc.paired(b1)
print(b2)
# Diagnostic accuracy of test 'y1'
#
# (Estimates, standard errors and 95%-confidence intervals)
#
# Est. SE Lower CL Upper CL
# Sensitivity 0.8802661 0.01528718 0.8503038 0.9102284
# Specificity 0.6781609 0.02891782 0.6214830 0.7348388
# PPV 0.8253638 0.01731081 0.7914353 0.8592924
# NPV 0.7662338 0.02784617 0.7116563 0.8208113
#
# Est. SE (log) Lower CL Upper CL
# PDLR 2.7351124 0.0915147 2.2860079 3.2724472
# NDLR 0.1765568 0.1346088 0.1356142 0.2298601
#
# ----------------------------------------------------------
# Diagnostic accuracy of test 'y2'
#
# (Estimates, standard errors and 95%-confidence intervals)
#
# Est. SE Lower CL Upper CL
# Sensitivity 0.7560976 0.02022128 0.7164646 0.7957305
# Specificity 0.7969349 0.02490054 0.7481307 0.8457390
# PPV 0.8654822 0.01718980 0.8317908 0.8991736
# NPV 0.6540881 0.02667395 0.6018081 0.7063680
#
# Est. SE (log) Lower CL Upper CL
# PDLR 3.7234238 0.1255060 2.9114648 4.7618247
# NDLR 0.3060507 0.0885996 0.2572629 0.3640906
Compare predictive values
Test based on weighted generalized score statistic:
pv.wgs(b1)
# $ppv
# test1 test2 diff test.statistic p.value
# 0.82536383 0.86548223 0.04011841 5.46588745 0.01939120
#
# $npv
# test1 test2 diff test.statistic p.value
# 7.662338e-01 6.540881e-01 -1.121457e-01 1.653540e+01 4.775012e-05
#
# $method
# [1] "weighted generalized score statistic (wgs)"
Estimation and test of relative predictive values:
pv.rpv(b1)
# $ppv
# test1 test2 rppv se.log.rppv lcl.rppv
# 0.82536383 0.86548223 1.04860694 0.01991247 1.00847050
# ucl.rppv test.statistic p.value
# 1.09034078 2.38355929 0.01714612
#
# $npv
# test1 test2 rnpv se.log.rnpv lcl.rnpv
# 7.662338e-01 6.540881e-01 8.536403e-01 3.783679e-02 7.926258e-01
# ucl.rnpv test.statistic p.value
# 9.193516e-01 -4.182314e+00 2.885568e-05
#
# $Sigma
# log.rppv log.rnpv
# log.rppv 0.0003965065 0.0004024578
# log.rnpv 0.0004024578 0.0014316223
#
# $method
# [1] "relative predictive values (rpv)"
#
# $alpha
# [1] 0.05
Citing DTComPair
citation("DTComPair")
# To cite DTComPair in publications use:
#
# Stock C, Hielscher T, Discacciati A (2023). DTComPair: comparison of
# binary diagnostic tests in a paired study design. R package, version
# 1.2.2. URL: https://CRAN.R-project.org/package=DTComPair.
#
# A BibTeX entry for LaTeX users is
#
# @Misc{,
# title = {{DTComPair}: comparison of binary diagnostic tests in a paired study design},
# author = {Christian Stock and Thomas Hielscher and Andrea Discacciati},
# year = {2023},
# note = {{R} package, version 1.2.2. {URL}: {https://CRAN.R-project.org/package=DTComPair}.},
# encoding = {UTF-8},
# }