Cardiovascular Risk Scores Calculator.
RiskScorescvd
Chest pain? Calculate your cardiovascular risk score.
The goal of RiskScorescvd r package is to calculate the most commonly used cardiovascular risk scores.
We have developed five of the most commonly used risk scores with a dependency (ASCVD [PooledCohort]) making the following available:
- HEART
- EDACS
- GRACE 2.0
- TIMI
- SCORE2/OP
- ASCVD
NOTE: Troponin I values should be used. Additional functions for Troponin T are under development
Installation
You can install from CRAN with:
# Install from CRAN
install.packages("RiskScorescvd")
You can install the development version of RiskScorescvd from GitHub with:
# install.packages("devtools")
devtools::install_github("dvicencio/RiskScorescvd")
Required variables for each risk score
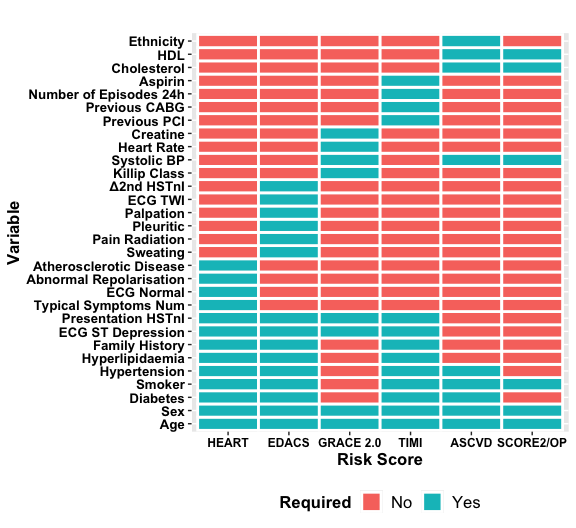
Example
This is a basic example of how the data set should look to calculate all risk scores available in the package:
library(RiskScorescvd)
#> Loading required package: PooledCohort
# Create a data frame or list with the necessary variables
# Set the number of rows
num_rows <- 100
# Create a large dataset with 100 rows
cohort_xx <- data.frame(
typical_symptoms.num = as.numeric(sample(0:6, num_rows, replace = TRUE)),
ecg.normal = as.numeric(sample(c(0, 1), num_rows, replace = TRUE)),
abn.repolarisation = as.numeric(sample(c(0, 1), num_rows, replace = TRUE)),
ecg.st.depression = as.numeric(sample(c(0, 1), num_rows, replace = TRUE)),
Age = as.numeric(sample(30:80, num_rows, replace = TRUE)),
diabetes = sample(c(1, 0), num_rows, replace = TRUE),
smoker = sample(c(1, 0), num_rows, replace = TRUE),
hypertension = sample(c(1, 0), num_rows, replace = TRUE),
hyperlipidaemia = sample(c(1, 0), num_rows, replace = TRUE),
family.history = sample(c(1, 0), num_rows, replace = TRUE),
atherosclerotic.disease = sample(c(1, 0), num_rows, replace = TRUE),
presentation_hstni = as.numeric(sample(10:100, num_rows, replace = TRUE)),
Gender = sample(c("male", "female"), num_rows, replace = TRUE),
sweating = as.numeric(sample(c(0, 1), num_rows, replace = TRUE)),
pain.radiation = as.numeric(sample(c(0, 1), num_rows, replace = TRUE)),
pleuritic = as.numeric(sample(c(0, 1), num_rows, replace = TRUE)),
palpation = as.numeric(sample(c(0, 1), num_rows, replace = TRUE)),
ecg.twi = as.numeric(sample(c(0, 1), num_rows, replace = TRUE)),
second_hstni = as.numeric(sample(1:200, num_rows, replace = TRUE)),
killip.class = as.numeric(sample(1:4, num_rows, replace = TRUE)),
systolic.bp = as.numeric(sample(40:300, num_rows, replace = TRUE)),
heart.rate = as.numeric(sample(0:300, num_rows, replace = TRUE)),
creat = as.numeric(sample(0:4, num_rows, replace = TRUE)),
cardiac.arrest = as.numeric(sample(c(0, 1), num_rows, replace = TRUE)),
previous.pci = as.numeric(sample(c(0, 1), num_rows, replace = TRUE)),
previous.cabg = as.numeric(sample(c(0, 1), num_rows, replace = TRUE)),
aspirin = as.numeric(sample(c(0, 1), num_rows, replace = TRUE)),
number.of.episodes.24h = as.numeric(sample(0:20, num_rows, replace = TRUE)),
total.chol = as.numeric(sample(5:100, num_rows, replace = TRUE)),
total.hdl = as.numeric(sample(2:5, num_rows, replace = TRUE)),
Ethnicity = sample(c("white", "black", "asian", "other"), num_rows, replace = TRUE)
)
str(cohort_xx)
#> 'data.frame': 100 obs. of 31 variables:
#> $ typical_symptoms.num : num 2 2 0 5 3 4 5 4 4 0 ...
#> $ ecg.normal : num 0 1 1 1 1 1 1 0 1 0 ...
#> $ abn.repolarisation : num 0 0 0 0 0 0 1 0 1 0 ...
#> $ ecg.st.depression : num 0 0 1 1 0 1 0 0 1 1 ...
#> $ Age : num 52 39 41 44 40 80 77 43 80 61 ...
#> $ diabetes : num 0 0 0 0 0 0 0 0 0 1 ...
#> $ smoker : num 1 0 1 1 1 0 0 1 0 1 ...
#> $ hypertension : num 1 0 0 1 1 1 1 1 1 0 ...
#> $ hyperlipidaemia : num 1 0 1 1 1 0 0 0 0 0 ...
#> $ family.history : num 0 1 0 0 0 0 1 1 0 1 ...
#> $ atherosclerotic.disease: num 1 1 0 0 1 1 0 1 1 0 ...
#> $ presentation_hstni : num 72 72 59 49 93 56 65 49 63 55 ...
#> $ Gender : chr "female" "male" "female" "male" ...
#> $ sweating : num 1 1 0 0 1 1 0 1 0 0 ...
#> $ pain.radiation : num 0 0 1 0 1 1 1 0 1 1 ...
#> $ pleuritic : num 1 0 0 0 0 0 1 1 0 1 ...
#> $ palpation : num 1 0 0 1 1 1 1 1 0 1 ...
#> $ ecg.twi : num 1 1 1 1 0 1 0 0 0 0 ...
#> $ second_hstni : num 144 81 100 189 143 153 167 67 177 148 ...
#> $ killip.class : num 4 4 4 1 3 2 4 3 2 1 ...
#> $ systolic.bp : num 105 255 141 128 120 145 179 269 283 90 ...
#> $ heart.rate : num 296 119 13 121 84 140 72 154 107 200 ...
#> $ creat : num 4 1 3 3 1 2 2 0 4 1 ...
#> $ cardiac.arrest : num 0 0 1 0 0 1 1 1 0 1 ...
#> $ previous.pci : num 1 0 0 0 1 1 1 0 1 0 ...
#> $ previous.cabg : num 1 0 1 1 1 1 0 1 1 1 ...
#> $ aspirin : num 0 0 0 0 0 0 1 1 1 1 ...
#> $ number.of.episodes.24h : num 11 2 2 2 6 16 10 13 6 20 ...
#> $ total.chol : num 84 81 62 99 21 69 25 86 17 69 ...
#> $ total.hdl : num 3 3 2 4 4 2 5 2 5 3 ...
#> $ Ethnicity : chr "black" "asian" "other" "black" ...
Calculation and Results
This is a basic example of how to calculate all risk scores available in the package and create a new data set with 12 new variables of the calculated and classified risk scores:
# Call the function with the cohort_xx to calculate all risk scores available in the package
new_data_frame <- calc_scores(data = cohort_xx)
# Select columns created after calculation
All_scores <- new_data_frame %>% select(HEART_score, HEART_strat, EDACS_score, EDACS_strat, GRACE_score, GRACE_strat, TIMI_score, TIMI_strat, SCORE2_score, SCORE2_strat, ASCVD_score, ASCVD_strat)
# Observe the results
head(All_scores)
#> # A tibble: 6 × 12
#> # Rowwise:
#> HEART_score HEART_strat EDACS_score EDACS_strat GRACE_score GRACE_strat
#> <dbl> <ord> <dbl> <ord> <dbl> <ord>
#> 1 7 High risk -1 Not low risk 154 High risk
#> 2 4 Moderate risk 11 Not low risk 77 Low risk
#> 3 5 Moderate risk 7 Not low risk 105 Moderate risk
#> 4 7 High risk 6 Not low risk 84 Low risk
#> 5 4 Moderate risk 14 Not low risk 82 Low risk
#> 6 9 High risk 18 Not low risk 164 High risk
#> # ℹ 6 more variables: TIMI_score <dbl>, TIMI_strat <ord>, SCORE2_score <dbl>,
#> # SCORE2_strat <ord>, ASCVD_score <dbl>, ASCVD_strat <ord>
# Create a summary of them to obtain an initial idea of distribution
summary(All_scores)
#> HEART_score HEART_strat EDACS_score EDACS_strat
#> Min. : 3.00 Low risk : 2 Min. :-6.00 Low risk : 0
#> 1st Qu.: 6.00 Moderate risk:43 1st Qu.: 6.00 Not low risk:100
#> Median : 7.00 High risk :55 Median :11.00
#> Mean : 6.54 Mean :11.29
#> 3rd Qu.: 7.00 3rd Qu.:18.00
#> Max. :10.00 Max. :30.00
#> GRACE_score GRACE_strat TIMI_score TIMI_strat
#> Min. : 33.0 Low risk :24 Min. :2.00 Very low risk: 0
#> 1st Qu.: 90.0 Moderate risk:27 1st Qu.:4.00 Low risk : 4
#> Median :117.5 High risk :49 Median :4.00 Moderate risk:48
#> Mean :116.1 Mean :4.45 High risk :48
#> 3rd Qu.:143.2 3rd Qu.:5.00
#> Max. :184.0 Max. :7.00
#> SCORE2_score SCORE2_strat ASCVD_score ASCVD_strat
#> Min. : 0.00 Very low risk: 0 Min. :0.0000 Very low risk:19
#> 1st Qu.: 20.00 Low risk :10 1st Qu.:0.1300 Low risk : 1
#> Median : 90.00 Moderate risk: 6 Median :0.4400 Moderate risk:15
#> Mean : 65.85 High risk :84 Mean :0.4742 High risk :65
#> 3rd Qu.:100.00 3rd Qu.:0.8500
#> Max. :100.00 Max. :1.0000