Description
Bayesian Estimation and Optimisation of Expected False Discovery Rate.
Description
Implements the Bayesian FDR control described by Newton et al. (2004), <doi:10.1093/biostatistics/5.2.155>. Allows optimisation and visualisation of expected error rates based on tail posterior probability tests. Based on code written by Catalina Vallejos for BASiCS, see Beyond comparisons of means: understanding changes in gene expression at the single-cell level Vallejos et al. (2016) <doi:10.1186/s13059-016-0930-3>.
README.md
bayefdr
The goal of bayefdr is to provide tools for the estimation and optimisation of Bayesian expected false discovery and expected false negative rates.
Installation
You can install the released version of bayefdr from CRAN with:
install.packages("bayefdr")
# development version:
## devtools::install_github("VallejosGroup/bayefdr")
Usage
The main functions in this package are efdr
, efnr
and efdr_search
. efdr
and efnr
calculate the EFDR or EFNR for a vector of probabilities given a specified probability threshold. efdr_search
finds the probability threshold that matches a target EFDR as closely as possible. The basic input to this function is a vector of probabilities and a target EFDR.
library("bayefdr")
set.seed(42)
probs <- runif(100)
efdr(0.7, probs)
#> [1] 0.1429126
efnr(0.7, probs)
#> [1] 0.3531349
efdr <- efdr_search(probs, target_efdr = 0.1)
efdr
#> An object of class 'bayefdr'.
#> Optimal threshold: 0.768 EFDR: 0.0985 EFNR: 0.399
The output of this function is a data.frame
with some extra attributes. There is a plot method too.
head(efdr)
#> threshold EFDR EFNR
#> 1 0.50000 0.239581 0.2361073
#> 2 0.50025 0.239581 0.2361073
#> 3 0.50050 0.239581 0.2361073
#> 4 0.50075 0.239581 0.2361073
#> 5 0.50100 0.239581 0.2361073
#> 6 0.50125 0.239581 0.2361073
plot(efdr)
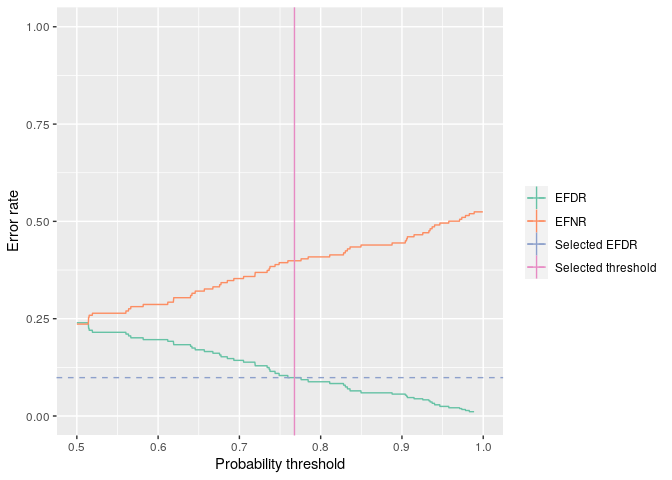