Bayesian Change-Point Detection for Process Monitoring with Fault Detection.
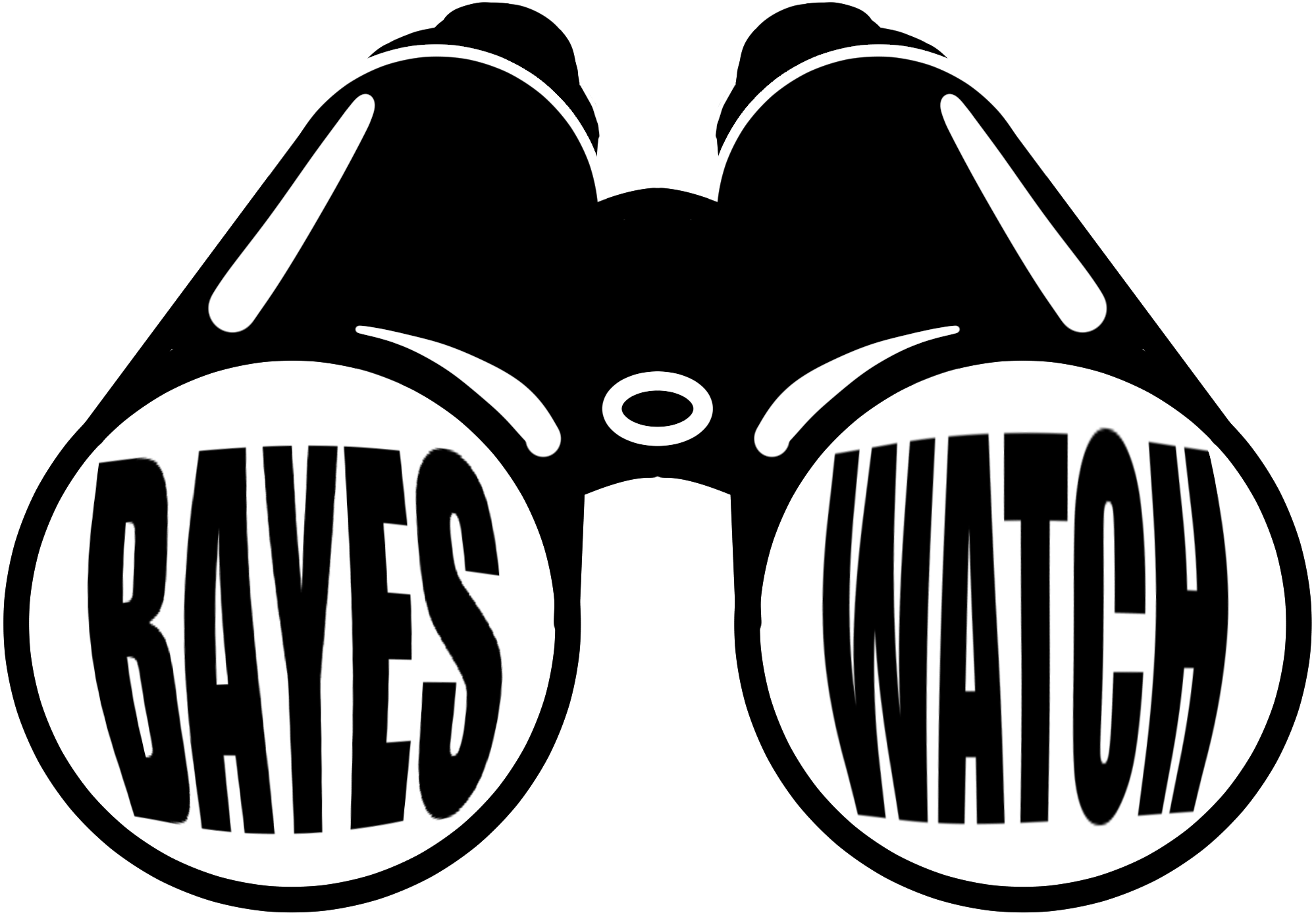
The main method bayeswatch
of this package fits an array of Gaussian Graphical Mixture Models to groupings of homogeneous data in time, called regimes, which we model as the observed states of a Markov process with unknown transition probabilities. While the primary goal of this model is to identify when there is a regime change, as this indicates a significant change in input data distribution, an attractive consequence of this approach is a rich collection of models fit to many different possible regimes. A fault detection system that leverages these model fits to describe the cause of a regime change is included in detect_faults
. For further technical details on these methods, see the Citations section.
This repository is organized as a stand-alone R package. For questions, issues, or clarifications please reach out to Murph: [email protected]. Feel free to email any applications; we'd be happy to highlight them here.
Installation
You can install the latest version from CRAN using:
install.packages("bayesWatch")
require(bayesWatch)
Examples
Simulated data are available with a change-point imposed after day 5. This change-point only occurs for variables 3 and 4, with 4 seeing the more significant change.
data("full_data")
data("day_of_observations")
data("day_dts")
my_fit = bayeswatch(full_data, day_of_observations, day_dts,
iterations = 500, g.prior = 1, linger_parameter = 20, n.cores=3,
wishart_df_inital = 3, hyperprior_b = 3, lambda = 5)
MCMC chain running...
# 5->10->15->20->25->30->35->40->45->50->55->60->65->70->75->80->85->90->95->100
# [1] "MCMC sampling complete. Performing fault detection calculations..."
print(my_fit)
# bayesWatch object
# ----------------------------------
# Time-point Change-point probability
# 1 1 0.000000000
# 2 2 0.000000000
# 3 3 0.000000000
# 4 4 0.023904382
# 5 5 0.972111554
# 6 6 0.055776892
# 7 7 0.003984064
# 8 8 0.011952191
# 9 9 0.000000000
plot(my_fit)
Once the regime vector is fit, we can print out the fault detection graphs.
detect_faults(my_fit)
Packages Required
ggplot2, gridExtra, parallel, Rcpp, Matrix, CholWishart, Hotelling, MASS, ess, stats, methods, BDgraph
Citation
Alexander C. Murph, Curtis B. Storlie, Patrick M. Wilson, Jonathan P. Williams, & Jan Hannig. (2023). Bayes Watch: Bayesian Change-point Detection for Process Monitoring with Fault Detection.