Cross-Validating Regression Models.
cv package for R: Cross-Validating Regression Models
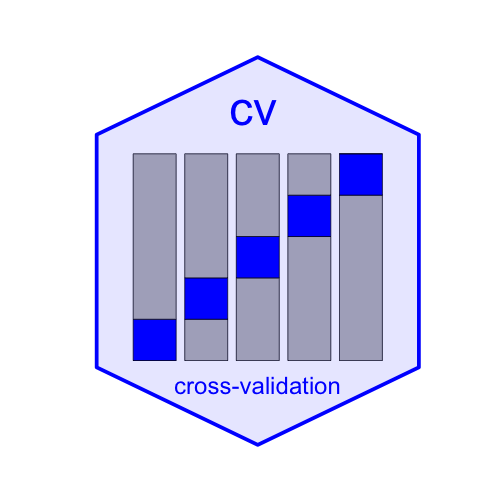
The cv package for R provides a consistent and extensible framework for cross-validating standard R statistical models. Some of the functions supplied by the package:
cv()
is a generic function with a default method, computationally efficient"lm"
and"glm"
methods, an"rlm"
method (for robust linear models), and a method for a list of competing models. There are also"merMod"
,"lme"
, and"glmmTMB"
methods for mixed-effects models.cv()
supports parallel computations.mse()
(mean-squared error),rmse()
(root-mean-squared error),medAbsErr()
(median absolute error), andBayesRule()
are cross-validation criteria ("cost functions"), suitable for use withcv()
.cv()
also can cross-validate a selection procedure (such as the following) for a regression model:cvModelList()
employs CV to select a model from among a number of candidates, and then cross-validates this model-selection procedure.selectStepAIC()
is a predictor-selection procedure based on thestepAIC()
function in the MASS package.selectTrans()
is a procedure for selecting predictor and response transformations in regression, based on thepowerTransform()
function in the car package.selectTransStepAIC()
is a procedure that first selects predictor and response transformations and then selects predictors.
For additional introductory information on using the cv package, see the "Cross-validating regression models" vignette (vignette("cv", package="cv")
). There are also vignettes on cross-validating mixed-effects models (vignette("cv-mixed", package="cv")
), cross-validating model selection (vignette("cv-selection", package="cv")
), and computational and technical notes (vignette("cv-notes", package="cv")
). The cv package is designed to be extensible to other classes of regression models, other CV criteria, and other model-selection procedures; for details, see the "Extending the cv package" vignette (vignette("cv-extend", package="cv")
).
Installing the cv package
To install the current version of the cv package from CRAN:
install.packages("cv")
To install the development version of the cv package from GitHub:
if (!require(remotes)) install.packages("remotes")
remotes::install_github("gmonette/cv", build_vignettes=TRUE,
dependencies=TRUE)