Description
Ecological Analysis and Visualization.
Description
Support ecological analyses such as ordination and clustering. Contains consistent and easy wrapper functions of 'stat', 'vegan', and 'labdsv' packages, and visualisation functions of ordination and clustering.
README.md
ecan
The goal of ecan is to support ecological analysis.
Installation
install.packages("ecan")
# development
# install.packages("devtools")
devtools::install_github("matutosi/ecan")
You can use almost the same functionality in shiny.
https://matutosi.shinyapps.io/ecanvis/ .
Example
Prepare and convert data
library(ecan)
library(vegan)
#> Loading required package: permute
#> Loading required package: lattice
#> This is vegan 2.6-4
library(dplyr)
#>
#> Attaching package: 'dplyr'
#> The following objects are masked from 'package:stats':
#>
#> filter, lag
#> The following objects are masked from 'package:base':
#>
#> intersect, setdiff, setequal, union
library(stringr)
library(tibble)
library(ggplot2)
data(dune)
data(dune.env)
df <-
table2df(dune) %>%
dplyr::left_join(tibble::rownames_to_column(dune.env, "stand"))
#> Joining with `by = join_by(stand)`
sp_dammy <-
tibble::tibble("species" = colnames(dune),
"dammy_1" = stringr::str_sub(colnames(dune), 1, 1),
"dammy_6" = stringr::str_sub(colnames(dune), 6, 6))
df <-
df %>%
dplyr::left_join(sp_dammy)
#> Joining with `by = join_by(species)`
df
#> # A tibble: 197 × 10
#> stand species abundance A1 Moisture Manage…¹ Use Manure dammy_1 dammy_6
#> <chr> <chr> <dbl> <dbl> <ord> <fct> <ord> <ord> <chr> <chr>
#> 1 1 Achimill 1 2.8 1 SF Hayp… 4 A i
#> 2 1 Elymrepe 4 2.8 1 SF Hayp… 4 E e
#> 3 1 Lolipere 7 2.8 1 SF Hayp… 4 L e
#> 4 1 Poaprat 4 2.8 1 SF Hayp… 4 P a
#> 5 1 Poatriv 2 2.8 1 SF Hayp… 4 P i
#> 6 2 Achimill 3 3.5 1 BF Hayp… 2 A i
#> 7 2 Alopgeni 2 3.5 1 BF Hayp… 2 A e
#> 8 2 Bellpere 3 3.5 1 BF Hayp… 2 B e
#> 9 2 Bromhord 4 3.5 1 BF Hayp… 2 B o
#> 10 2 Elymrepe 4 3.5 1 BF Hayp… 2 E e
#> # … with 187 more rows, and abbreviated variable name ¹Management
Diversity index
div <-
shdi(df) %>%
dplyr::left_join(select_one2multi(df, "stand"))
#> Joining with `by = join_by(stand)`
group <- "Management"
div_index <- "s"
div %>%
ggplot(aes(x = .data[[group]], y = .data[[div_index]])) +
geom_boxplot(outlier.shape = NA) + # do not show outer point
geom_jitter(height = 0, width = 0.1)
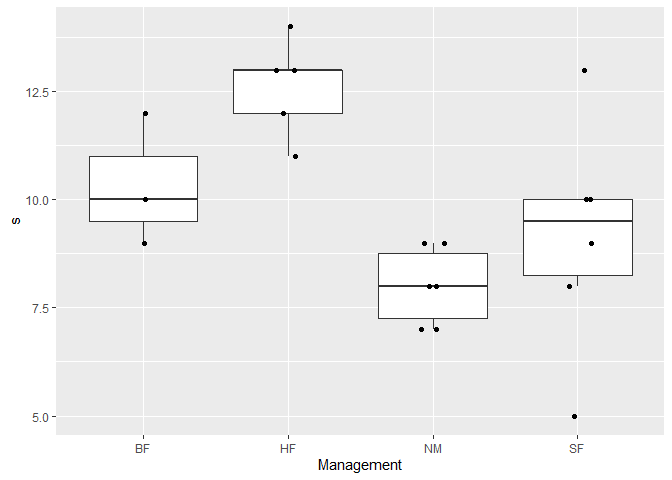
Indicator Species Analysis (ISA, ind val)
ind_val(df, group = "Moisture", row_data = TRUE)
#> $relfrq
#> 1 2 3 4
#> Achimill 0.7142857 0.50 0.0000000 0.0
#> Elymrepe 0.4285714 0.50 0.0000000 0.5
#> Lolipere 1.0000000 0.75 0.1428571 0.5
#> Poaprat 1.0000000 1.00 0.2857143 0.5
#> Poatriv 0.7142857 0.75 0.4285714 1.0
#> Alopgeni 0.1428571 0.50 0.4285714 1.0
#> Bellpere 0.4285714 0.75 0.0000000 0.0
#> Bromhord 0.4285714 0.50 0.0000000 0.0
#> Scorautu 0.8571429 1.00 0.8571429 1.0
#> Trifrepe 0.8571429 0.75 0.7142857 1.0
#> Agrostol 0.0000000 0.50 0.8571429 1.0
#> Bracruta 0.7142857 0.75 0.7142857 1.0
#> Cirsarve 0.0000000 0.25 0.0000000 0.0
#> Sagiproc 0.1428571 0.25 0.4285714 1.0
#> Anthodor 0.4285714 0.50 0.1428571 0.0
#> Planlanc 0.7142857 0.50 0.0000000 0.0
#> Rumeacet 0.4285714 0.00 0.0000000 1.0
#> Trifprat 0.4285714 0.00 0.0000000 0.0
#> Juncbufo 0.1428571 0.00 0.1428571 1.0
#> Eleopalu 0.0000000 0.00 0.7142857 0.0
#> Juncarti 0.0000000 0.00 0.5714286 0.5
#> Ranuflam 0.0000000 0.00 0.8571429 0.0
#> Vicilath 0.2857143 0.25 0.0000000 0.0
#> Hyporadi 0.1428571 0.25 0.1428571 0.0
#> Chenalbu 0.0000000 0.00 0.1428571 0.0
#> Comapalu 0.0000000 0.00 0.2857143 0.0
#> Callcusp 0.0000000 0.00 0.4285714 0.0
#> Airaprae 0.0000000 0.25 0.1428571 0.0
#> Salirepe 0.1428571 0.00 0.2857143 0.0
#> Empenigr 0.0000000 0.00 0.1428571 0.0
#>
#> $relabu
#> 1 2 3 4
#> Achimill 0.48780488 0.5121951 0.00000000 0.00000000
#> Elymrepe 0.25531915 0.2978723 0.00000000 0.44680851
#> Lolipere 0.46204620 0.3927393 0.05280528 0.09240924
#> Poaprat 0.35036496 0.3576642 0.08759124 0.20437956
#> Poatriv 0.24806202 0.2713178 0.15503876 0.32558140
#> Alopgeni 0.02846975 0.2241993 0.19928826 0.54804270
#> Bellpere 0.40000000 0.6000000 0.00000000 0.00000000
#> Bromhord 0.39506173 0.6049383 0.00000000 0.00000000
#> Scorautu 0.33922261 0.2226148 0.24028269 0.19787986
#> Trifrepe 0.27636364 0.2290909 0.18909091 0.30545455
#> Agrostol 0.00000000 0.2818792 0.38926174 0.32885906
#> Bracruta 0.29197080 0.1532847 0.24817518 0.30656934
#> Cirsarve 0.00000000 1.0000000 0.00000000 0.00000000
#> Sagiproc 0.05161290 0.2258065 0.18064516 0.54193548
#> Anthodor 0.33333333 0.5185185 0.14814815 0.00000000
#> Planlanc 0.70588235 0.2941176 0.00000000 0.00000000
#> Rumeacet 0.50000000 0.0000000 0.00000000 0.50000000
#> Trifprat 1.00000000 0.0000000 0.00000000 0.00000000
#> Juncbufo 0.06060606 0.0000000 0.09090909 0.84848485
#> Eleopalu 0.00000000 0.0000000 1.00000000 0.00000000
#> Juncarti 0.00000000 0.0000000 0.50000000 0.50000000
#> Ranuflam 0.00000000 0.0000000 1.00000000 0.00000000
#> Vicilath 0.63157895 0.3684211 0.00000000 0.00000000
#> Hyporadi 0.19047619 0.3333333 0.47619048 0.00000000
#> Chenalbu 0.00000000 0.0000000 1.00000000 0.00000000
#> Comapalu 0.00000000 0.0000000 1.00000000 0.00000000
#> Callcusp 0.00000000 0.0000000 1.00000000 0.00000000
#> Airaprae 0.00000000 0.5384615 0.46153846 0.00000000
#> Salirepe 0.27272727 0.0000000 0.72727273 0.00000000
#> Empenigr 0.00000000 0.0000000 1.00000000 0.00000000
#>
#> $indval
#> 1 2 3 4
#> Achimill 0.348432056 0.25609756 0.000000000 0.00000000
#> Elymrepe 0.109422492 0.14893617 0.000000000 0.22340426
#> Lolipere 0.462046205 0.29455446 0.007543612 0.04620462
#> Poaprat 0.350364964 0.35766423 0.025026069 0.10218978
#> Poatriv 0.177187154 0.20348837 0.066445183 0.32558140
#> Alopgeni 0.004067107 0.11209964 0.085409253 0.54804270
#> Bellpere 0.171428571 0.45000000 0.000000000 0.00000000
#> Bromhord 0.169312169 0.30246914 0.000000000 0.00000000
#> Scorautu 0.290762241 0.22261484 0.205956588 0.19787986
#> Trifrepe 0.236883117 0.17181818 0.135064935 0.30545455
#> Agrostol 0.000000000 0.14093960 0.333652924 0.32885906
#> Bracruta 0.208550574 0.11496350 0.177267987 0.30656934
#> Cirsarve 0.000000000 0.25000000 0.000000000 0.00000000
#> Sagiproc 0.007373272 0.05645161 0.077419355 0.54193548
#> Anthodor 0.142857143 0.25925926 0.021164021 0.00000000
#> Planlanc 0.504201681 0.14705882 0.000000000 0.00000000
#> Rumeacet 0.214285714 0.00000000 0.000000000 0.50000000
#> Trifprat 0.428571429 0.00000000 0.000000000 0.00000000
#> Juncbufo 0.008658009 0.00000000 0.012987013 0.84848485
#> Eleopalu 0.000000000 0.00000000 0.714285714 0.00000000
#> Juncarti 0.000000000 0.00000000 0.285714286 0.25000000
#> Ranuflam 0.000000000 0.00000000 0.857142857 0.00000000
#> Vicilath 0.180451128 0.09210526 0.000000000 0.00000000
#> Hyporadi 0.027210884 0.08333333 0.068027211 0.00000000
#> Chenalbu 0.000000000 0.00000000 0.142857143 0.00000000
#> Comapalu 0.000000000 0.00000000 0.285714286 0.00000000
#> Callcusp 0.000000000 0.00000000 0.428571429 0.00000000
#> Airaprae 0.000000000 0.13461538 0.065934066 0.00000000
#> Salirepe 0.038961039 0.00000000 0.207792208 0.00000000
#> Empenigr 0.000000000 0.00000000 0.142857143 0.00000000
#>
#> $maxcls
#> Achimill Elymrepe Lolipere Poaprat Poatriv Alopgeni Bellpere Bromhord
#> 1 4 1 2 4 4 2 2
#> Scorautu Trifrepe Agrostol Bracruta Cirsarve Sagiproc Anthodor Planlanc
#> 1 4 3 4 2 4 2 1
#> Rumeacet Trifprat Juncbufo Eleopalu Juncarti Ranuflam Vicilath Hyporadi
#> 4 1 4 3 3 3 1 2
#> Chenalbu Comapalu Callcusp Airaprae Salirepe Empenigr
#> 3 3 3 2 3 3
#>
#> $indcls
#> Achimill Elymrepe Lolipere Poaprat Poatriv Alopgeni Bellpere
#> 0.34843206 0.22340426 0.46204620 0.35766423 0.32558140 0.54804270 0.45000000
#> Bromhord Scorautu Trifrepe Agrostol Bracruta Cirsarve Sagiproc
#> 0.30246914 0.29076224 0.30545455 0.33365292 0.30656934 0.25000000 0.54193548
#> Anthodor Planlanc Rumeacet Trifprat Juncbufo Eleopalu Juncarti
#> 0.25925926 0.50420168 0.50000000 0.42857143 0.84848485 0.71428571 0.28571429
#> Ranuflam Vicilath Hyporadi Chenalbu Comapalu Callcusp Airaprae
#> 0.85714286 0.18045113 0.08333333 0.14285714 0.28571429 0.42857143 0.13461538
#> Salirepe Empenigr
#> 0.20779221 0.14285714
#>
#> $pval
#> Achimill Elymrepe Lolipere Poaprat Poatriv Alopgeni Bellpere Bromhord
#> 0.240 0.417 0.062 0.378 0.502 0.053 0.131 0.190
#> Scorautu Trifrepe Agrostol Bracruta Cirsarve Sagiproc Anthodor Planlanc
#> 0.797 0.698 0.383 0.614 0.286 0.072 0.311 0.107
#> Rumeacet Trifprat Juncbufo Eleopalu Juncarti Ranuflam Vicilath Hyporadi
#> 0.092 0.136 0.004 0.024 0.214 0.001 0.699 1.000
#> Chenalbu Comapalu Callcusp Airaprae Salirepe Empenigr
#> 1.000 0.449 0.072 0.755 0.594 1.000
#>
#> $error
#> [1] 0
#>
#> attr(,"class")
#> [1] "indval"
ind_val(df, group = "Management")
#> Joining with `by = join_by(numeric_Management)`
#> # A tibble: 30 × 4
#> Management species ind.val p.value
#> <fct> <chr> <dbl> <dbl>
#> 1 SF Elymrepe 0.188 0.684
#> 2 SF Alopgeni 0.547 0.038
#> 3 SF Agrostol 0.472 0.054
#> 4 SF Cirsarve 0.167 1
#> 5 SF Sagiproc 0.241 0.514
#> 6 SF Chenalbu 0.167 1
#> 7 BF Achimill 0.386 0.118
#> 8 BF Lolipere 0.45 0.07
#> 9 BF Poaprat 0.379 0.188
#> 10 BF Bellpere 0.362 0.126
#> # … with 20 more rows
ind_val(df, group = "Use")
#> Joining with `by = join_by(numeric_Use)`
#> # A tibble: 30 × 4
#> Use species ind.val p.value
#> <ord> <chr> <dbl> <dbl>
#> 1 Haypastu Elymrepe 0.292 0.288
#> 2 Haypastu Lolipere 0.259 0.796
#> 3 Haypastu Poaprat 0.288 0.824
#> 4 Haypastu Poatriv 0.451 0.118
#> 5 Haypastu Alopgeni 0.359 0.184
#> 6 Haypastu Agrostol 0.269 0.589
#> 7 Haypastu Cirsarve 0.125 1
#> 8 Haypastu Sagiproc 0.178 0.8
#> 9 Haypastu Juncbufo 0.118 0.848
#> 10 Haypastu Chenalbu 0.125 1
#> # … with 20 more rows
ind_val(df, group = "Manure")
#> Joining with `by = join_by(numeric_Manure)`
#> # A tibble: 30 × 4
#> Manure species ind.val p.value
#> <ord> <chr> <dbl> <dbl>
#> 1 4 Elymrepe 0.5 0.048
#> 2 4 Lolipere 0.351 0.211
#> 3 4 Poaprat 0.315 0.28
#> 4 4 Bellpere 0.248 0.469
#> 5 4 Cirsarve 0.333 0.279
#> 6 2 Achimill 0.309 0.262
#> 7 2 Poatriv 0.299 0.394
#> 8 2 Bromhord 0.173 0.703
#> 9 2 Anthodor 0.178 0.763
#> 10 2 Rumeacet 0.522 0.041
#> # … with 20 more rows
Cluster analysis
library(ggdendro)
library(dendextend)
#> Registered S3 method overwritten by 'dendextend':
#> method from
#> rev.hclust vegan
#>
#> ---------------------
#> Welcome to dendextend version 1.16.0
#> Type citation('dendextend') for how to cite the package.
#>
#> Type browseVignettes(package = 'dendextend') for the package vignette.
#> The github page is: https://github.com/talgalili/dendextend/
#>
#> Suggestions and bug-reports can be submitted at: https://github.com/talgalili/dendextend/issues
#> You may ask questions at stackoverflow, use the r and dendextend tags:
#> https://stackoverflow.com/questions/tagged/dendextend
#>
#> To suppress this message use: suppressPackageStartupMessages(library(dendextend))
#> ---------------------
#>
#> Attaching package: 'dendextend'
#> The following object is masked from 'package:ggdendro':
#>
#> theme_dendro
#> The following object is masked from 'package:permute':
#>
#> shuffle
#> The following object is masked from 'package:stats':
#>
#> cutree
cls <- cluster(dune, c_method = "average", d_method = "euclidean")
ggdendro::ggdendrogram(cls)
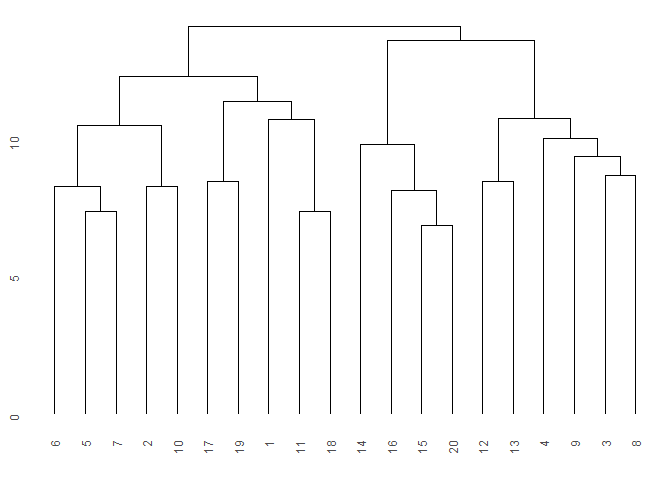
indiv <- "stand"
group <- "Use"
ggdendro::ggdendrogram(cls_add_group(cls, df, indiv, group))
#> Joining with `by = join_by(stand)`
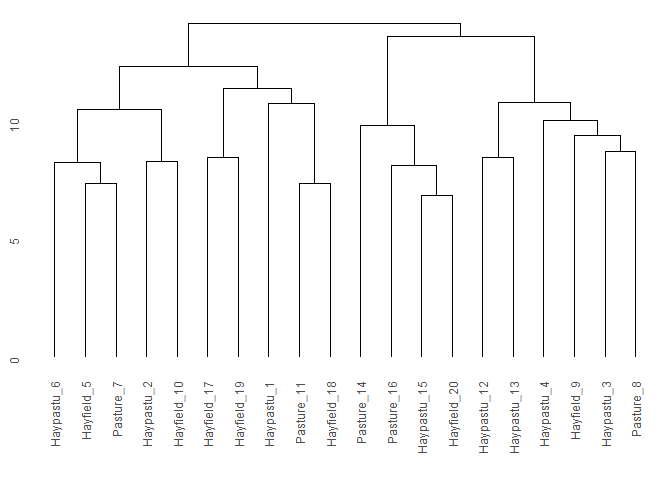
col <- cls_color(cls, df, indiv, group)
#> Joining with `by = join_by(stand)`
#> Joining with `by = join_by(Use)`
cls <-
cls_add_group(cls, df, indiv, group) %>%
stats::as.dendrogram()
#> Joining with `by = join_by(stand)`
labels_colors(cls) <- gray(0)
plot(cls)
dendextend::colored_bars(colors = col, cls, group, y_shift = 0, y_scale = 3)
par(new = TRUE)
plot(cls)
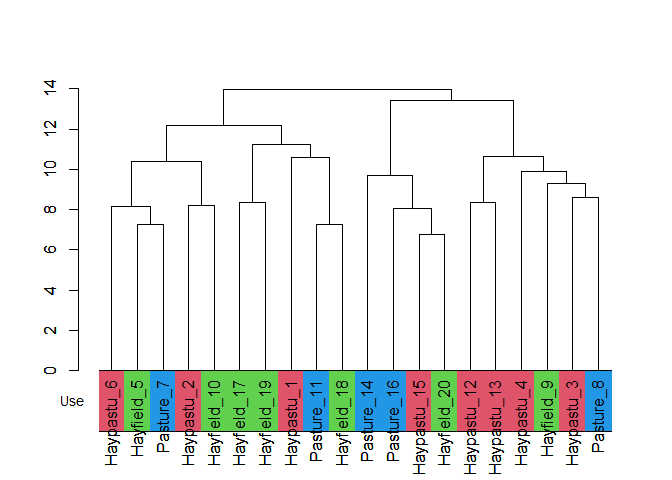
Ordination
ord_dca <- ordination(dune, o_method = "dca")
ord_pca <-
df %>%
df2table() %>%
ordination(o_method = "pca")
ord_dca_st <-
ord_extract_score(ord_dca, score = "st_scores")
ord_dca_st %>%
ggplot(aes(DCA1, DCA2, label = rownames(.))) +
geom_text()
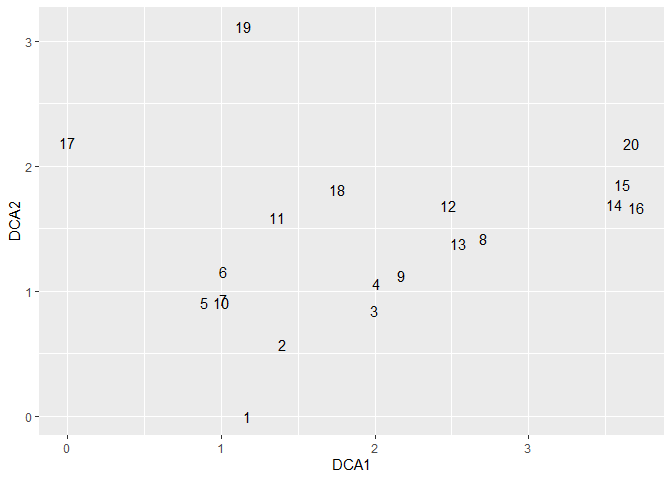
indiv <- "species"
group <- "dammy_1"
ord_pca_sp <-
ord_add_group(ord_pca, score = "sp_scores", df, indiv, group)
#> Joining with `by = join_by(species)`
ord_pca_sp %>%
ggplot(aes(PC1, PC2, label = rownames(.))) +
geom_point(aes(col = .data[[group]]), alpha = 0.4, size = 7) +
geom_text() +
theme_bw()
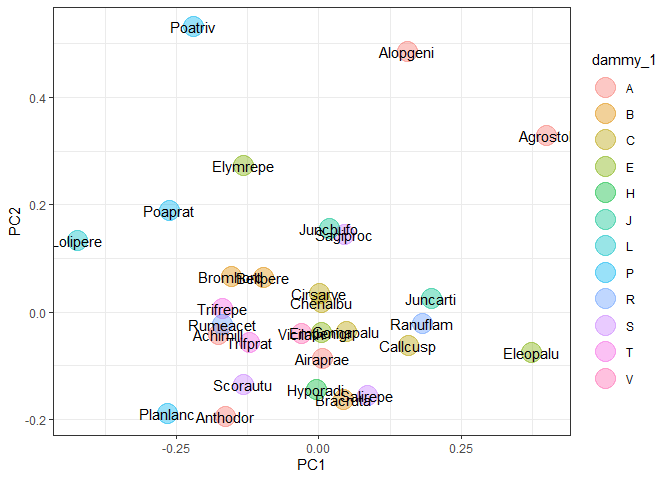
Citation
Toshikazu Matsumura (2022) Ecological analysis tools with R. https://github.com/matutosi/ecan/.