Description
Outlier Detection Tools for Functional Data Analysis.
Description
A collection of functions for outlier detection in functional data analysis. Methods implemented include directional outlyingness by Dai and Genton (2019) <doi:10.1016/j.csda.2018.03.017>, MS-plot by Dai and Genton (2018) <doi:10.1080/10618600.2018.1473781>, total variation depth and modified shape similarity index by Huang and Sun (2019) <doi:10.1080/00401706.2019.1574241>, and sequential transformations by Dai et al. (2020) <doi:10.1016/j.csda.2020.106960 among others. Additional outlier detection tools and depths for functional data like functional boxplot, (modified) band depth etc., are also available.
README.md
fdaoutlier
Outlier Detection Tools for Functional Data Analysis
`fdaoutlier` is a collection of outlier detectiontools for functional data analysis. Methods implemented include directional outlyingness, MS-plot, total variation depth, and sequential transformations among others.
Installation
You can install the current version of fdaoutliers from CRAN with:
install.packages("fdaoutlier")
or the latest the development version from GitHub with:
devtools::install_github("otsegun/fdaoutlier")
Example
Generate some functional data with magnitude outliers:
library(fdaoutlier)
simdata <- simulation_model1(plot = T, seed = 1)
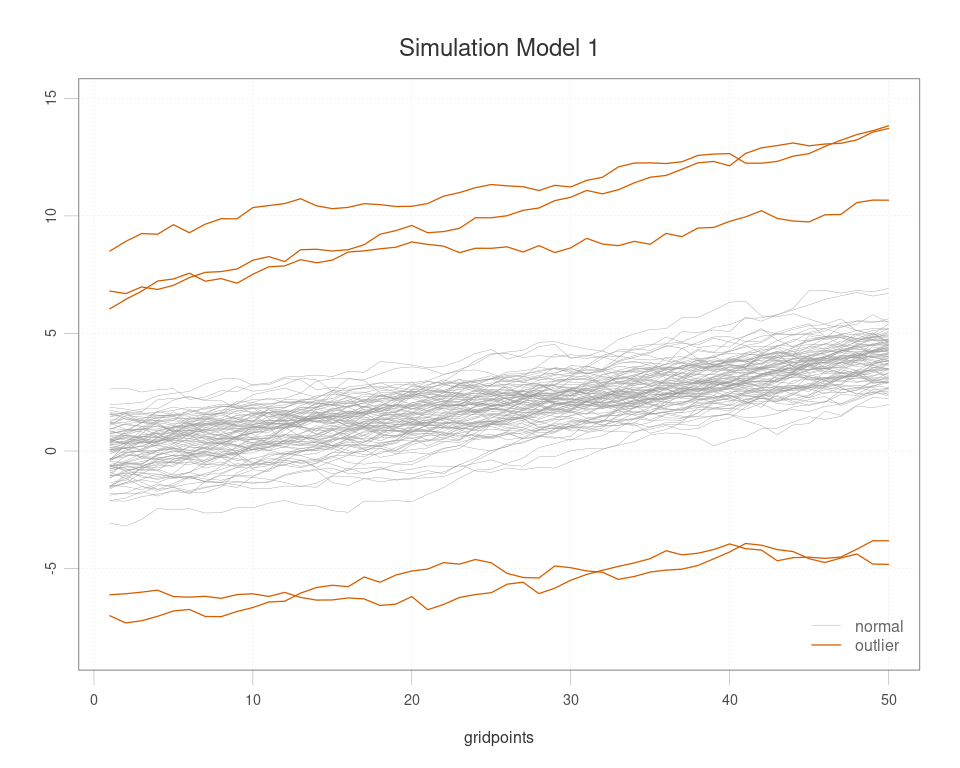
dim(simdata$data)
#> [1] 100 50
Next apply the msplot of Dai & Genton (2018)
ms <- msplot(simdata$data)
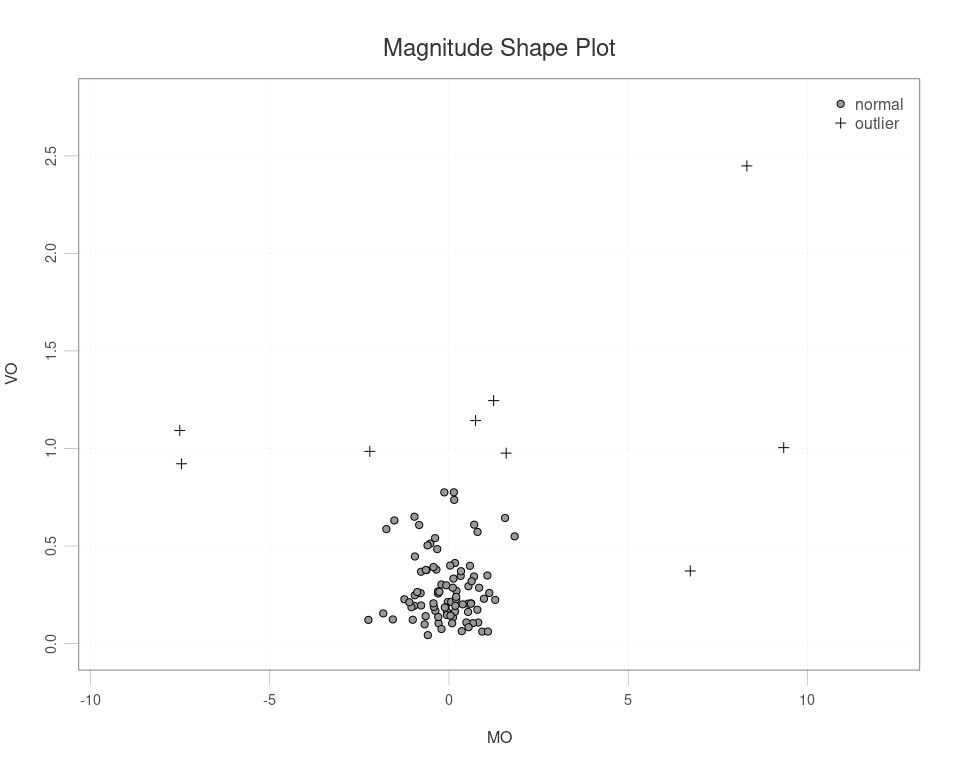
ms$outliers
#> [1] 4 7 17 26 29 55 62 66 76
simdata$true_outliers
#> [1] 4 7 17 55 66
Methods Implemented
- MS-Plot (Dai & Genton, 2018)
- TVDMSS (Huang & Sun, 2019)
- Extremal depth (Narisetty & Nair, 2016)
- Extreme rank length depth (Myllymäki et al., 2017; Dai et al., 2020)
- Directional quantile (Myllymäki et al., 2017; Dai et al., 2020)
- Fast band depth and modified band depth (Sun et al., 2012)
- Directional Outlyingness (Dai & Genton, 2019)
- Sequential transformation (Dai et al., 2020)
Bugs and Feature Requests
Kindly open an issue using Github issues.