High-Dimensional Aggregate Density Forecasts.
hdflex 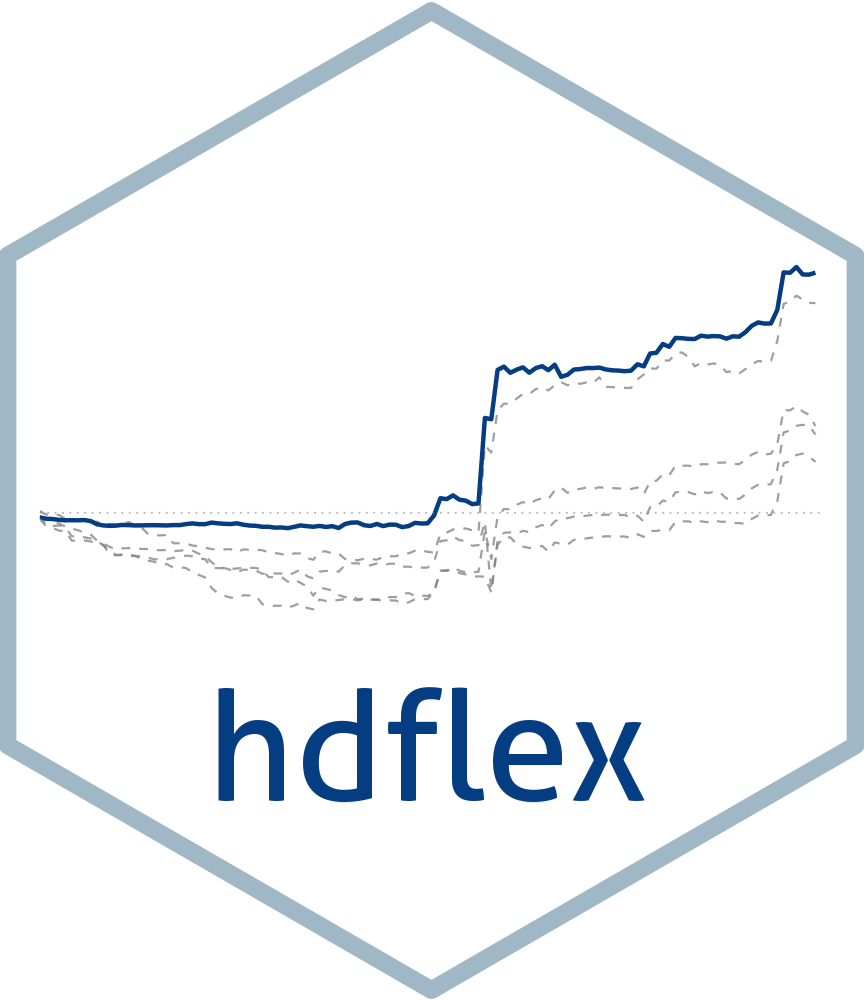
About
This package contains the forecasting algorithm developed by Adämmer, Lehmann and Schüssler (2023). Please cite the paper when using the package.
The package comprises four functions:
stsc()
can be used to directly apply the “Signal-Transform-Subset-Combination” forecasting algorithm described in Adämmer, Lehmann and Schüssler (2023).tvc()
can be used to compute density forecasts based on univariate time-varying coefficient (TV-C) models in state-space form (first part of the STSC algorithm).dsc()
can be used to dynamically generate forecast combinations from a subset of candidate density forecasts (second part of the STSC algorithm).summary_stsc()
returns a statistical summary for the forecasting results. It provides statistical measures such as Clark-West-Statistic, OOS-R2, Mean-Squared-Error and Cumulated Sum of Squared-Error-Differences.
Installation
You can install the released version of hdflex from CRAN:
install.packages("hdflex")
You can install hdflex from GitHub:
# install.packages("devtools")
devtools::install_github("https://github.com/lehmasve/hdflex")
The package compiles some C++ source code for installation, which is why you need the appropriate compilers:
On Windows you need Rtools available from CRAN.
On macOS you need the very least Xcode and a Fortran compiler - for more details see Compiler.
Usage
First example using the stsc()
function:
#########################################################
######### Forecasting quarterly U.S. inflation ##########
#### Please see Koop & Korobilis (2023) for further ####
#### details regarding the data & external forecasts ####
#########################################################
# Packages
library("hdflex")
########## Get Data ##########
# Load Data
inflation_data <- inflation_data
benchmark_ar2 <- benchmark_ar2
# Set Index for Target Variable
i <- 1 # (1 -> GDPCTPI; 2 -> PCECTPI; 3 -> CPIAUCSL; 4 -> CPILFESL)
# Subset Data (keep only data relevant for target variable i)
dataset <- inflation_data[, c(1+(i-1), # Target Variable
5+(i-1), # Lag 1
9+(i-1), # Lag 2
(13:16)[-i], # Remaining Price Series
17:452, # Exogenous Predictor Variables
seq(453+(i-1)*16,468+(i-1)*16))] # Ext. Point Forecasts
########## STSC ##########
# Set Target Variable
y <- dataset[, 1, drop = FALSE]
# Set 'Simple' Signals
X <- dataset[, 2:442, drop = FALSE]
# Set External Point Forecasts (Koop & Korobilis 2023)
F <- dataset[, 443:458, drop = FALSE]
# Set Dates
dates <- rownames(dataset)
# Set TV-C-Parameter
sample_length <- 4 * 5
lambda_grid <- c(0.90, 0.95, 1)
kappa_grid <- 0.98
# Set DSC-Parameter
gamma_grid <- c(0.40, 0.50, 0.60, 0.70, 0.80, 0.90,
0.91, 0.92, 0.93, 0.94, 0.95, 0.96, 0.97, 0.98, 0.99, 1.00)
psi_grid <- c(1:100)
delta <- 0.95
# Apply STSC-Function
results <- hdflex::stsc(y,
X,
F,
sample_length,
lambda_grid,
kappa_grid,
burn_in_tvc = 79,
gamma_grid,
psi_grid,
delta,
burn_in_dsc = 1,
method = 1,
equal_weight = TRUE,
risk_aversion = NULL,
min_weight = NULL,
max_weight = NULL)
# Assign STSC-Results
forecast_stsc <- results[[1]]
variance_stsc <- results[[2]]
chosen_gamma <- results[[3]]
chosen_psi <- results[[4]]
chosen_signals <- results[[5]]
# Define Evaluation Period (OOS-Period)
eval_date_start <- "1991-01-01"
eval_date_end <- "2021-12-31"
eval_period_idx <- which(dates > eval_date_start & dates <= eval_date_end)
# Trim Objects to Evaluation Period (OOS-Period)
oos_y <- y[eval_period_idx, ]
oos_forecast_stsc <- forecast_stsc[eval_period_idx]
oos_variance_stsc <- variance_stsc[eval_period_idx]
oos_chosen_gamma <- chosen_gamma[eval_period_idx]
oos_chosen_psi <- chosen_psi[eval_period_idx]
oos_chosen_signals <- chosen_signals[eval_period_idx, , drop = FALSE]
oos_dates <- dates[eval_period_idx]
# Add Dates
names(oos_forecast_stsc) <- oos_dates
names(oos_variance_stsc) <- oos_dates
names(oos_chosen_gamma) <- oos_dates
names(oos_chosen_psi) <- oos_dates
rownames(oos_chosen_signals) <- oos_dates
########## Evaluation ##########
# Apply Summary-Function
summary_results <- summary_stsc(oos_y,
benchmark_ar2[, i],
oos_forecast_stsc)
# Assign Summary-Results
cssed <- summary_results[[3]]
mse <- summary_results[[4]]
########## Visualization ##########
# Create CSSED-Plot
p1 <- plot(x = as.Date(oos_dates),
y = cssed,
ylim = c(-0.0008, 0.0008),
main = "Cumulated squared error differences",
type = "l",
lwd = 1.5,
xlab = "Date",
ylab = "CSSED") + abline(h = 0, lty = 2, col = "darkgray")
# Create Predictive Signals-Plot
vec <- seq_len(dim(oos_chosen_signals)[2])
mat <- oos_chosen_signals %*% diag(vec)
mat[mat == 0] <- NA
p2 <- matplot(x = as.Date(oos_dates),
y = mat,
cex = 0.4,
pch = 20,
type = "p",
main = "Evolution of selected signal(s)",
xlab = "Date",
ylab = "Predictive Signal")
# Create Psi-Plot
p3 <- plot(x = as.Date(oos_dates),
y = oos_chosen_psi,
ylim = c(1, 100),
main = "Evolution of the subset size",
type = "p",
cex = 0.75,
pch = 20,
xlab = "Date",
ylab = "Psi")
# Relative MSE
print(paste("Relative MSE:", round(mse[[1]] / mse[[2]], 4)))
# Print Plots
print(p1)
print(p2)
print(p3)
Second example using the tvc()
and dsc()
functions:
#########################################################
######### Forecasting quarterly U.S. inflation ##########
#### Please see Koop & Korobilis (2023) for further ####
#### details regarding the data & external forecasts ####
#########################################################
# Packages
library("hdflex")
########## Get Data ##########
# Load Data
inflation_data <- inflation_data
benchmark_ar2 <- benchmark_ar2
# Set Index for Target Variable
i <- 1 # (1 -> GDPCTPI; 2 -> PCECTPI; 3 -> CPIAUCSL; 4 -> CPILFESL)
# Subset Data (keep only data relevant for target variable i)
dataset <- inflation_data[, c(1+(i-1), # Target Variable
5+(i-1), # Lag 1
9+(i-1), # Lag 2
(13:16)[-i], # Remaining Price Series
17:452, # Exogenous Predictor Variables
seq(453+(i-1)*16,468+(i-1)*16))] # Ext. Point Forecasts
########## STSC ##########
### Part 1: TV-C Model ###
# Set Target Variable
y <- dataset[, 1, drop = FALSE]
# Set 'Simple' Signals
X <- dataset[, 2:442, drop = FALSE]
# Set External Point Forecasts (Koop & Korobilis 2023)
F <- dataset[, 443:458, drop = FALSE]
# Set TV-C-Parameter
sample_length <- 4 * 5
lambda_grid <- c(0.90, 0.95, 1)
kappa_grid <- 0.98
n_cores <- 4
# Apply TV-C-Function
results <- hdflex::tvc(y,
X,
F,
lambda_grid,
kappa_grid,
sample_length,
n_cores)
# Assign TV-C-Results
forecast_tvc <- results[[1]]
variance_tvc <- results[[2]]
# Define Burn-In Period
sample_period_idx <- 80:nrow(dataset)
sub_forecast_tvc <- forecast_tvc[sample_period_idx, , drop = FALSE]
sub_variance_tvc <- variance_tvc[sample_period_idx, , drop = FALSE]
sub_y <- y[sample_period_idx, , drop = FALSE]
sub_dates <- rownames(dataset)[sample_period_idx]
### Part 2: Dynamic Subset Combination ###
# Set DSC-Parameter
nr_mods <- ncol(sub_forecast_tvc)
gamma_grid <- c(0.40, 0.05, 0.60, 0.70, 0.80, 0.90,
0.91, 0.92, 0.93, 0.94, 0.95, 0.96, 0.97, 0.98, 0.99, 1.00)
psi_grid <- c(1:100)
delta <- 0.95
n_cores <- 4
# Apply DSC-Function
results <- hdflex::dsc(gamma_grid,
psi_grid,
sub_y,
sub_forecast_tvc,
sub_variance_tvc,
delta,
n_cores)
# Assign DSC-Results
sub_forecast_stsc <- results[[1]]
sub_variance_stsc <- results[[2]]
sub_chosen_gamma <- results[[3]]
sub_chosen_psi <- results[[4]]
sub_chosen_signals <- results[[5]]
# Define Evaluation Period (OOS-Period)
eval_date_start <- "1991-01-01"
eval_date_end <- "2021-12-31"
eval_period_idx <- which(sub_dates > eval_date_start & sub_dates <= eval_date_end)
# Trim Objects to Evaluation Period (OOS-Period)
oos_y <- sub_y[eval_period_idx, ]
oos_forecast_stsc <- sub_forecast_stsc[eval_period_idx]
oos_variance_stsc <- sub_variance_stsc[eval_period_idx]
oos_chosen_gamma <- sub_chosen_gamma[eval_period_idx]
oos_chosen_psi <- sub_chosen_psi[eval_period_idx]
oos_chosen_signals <- sub_chosen_signals[eval_period_idx, , drop = FALSE]
oos_dates <- sub_dates[eval_period_idx]
# Add Dates
names(oos_forecast_stsc) <- oos_dates
names(oos_variance_stsc) <- oos_dates
names(oos_chosen_gamma) <- oos_dates
names(oos_chosen_psi) <- oos_dates
rownames(oos_chosen_signals) <- oos_dates
### Part 3: Evaluation ###
# Apply Summary-Function
summary_results <- summary_stsc(oos_y,
benchmark_ar2[, i],
oos_forecast_stsc)
# Assign Summary-Results
cssed <- summary_results[[3]]
mse <- summary_results[[4]]
########## Visualization ##########
# Create CSSED-Plot
p1 <- plot(x = as.Date(oos_dates),
y = cssed,
ylim = c(-0.0008, 0.0008),
main = "Cumulated squared error differences",
type = "l",
lwd = 1.5,
xlab = "Date",
ylab = "CSSED") + abline(h = 0, lty = 2, col = "darkgray")
# Create Predictive Signals-Plot
vec <- seq_len(dim(oos_chosen_signals)[2])
mat <- oos_chosen_signals %*% diag(vec)
mat[mat == 0] <- NA
p2 <- matplot(x = as.Date(oos_dates),
y = mat,
cex = 0.4,
pch = 20,
type = "p",
main = "Evolution of selected signal(s)",
xlab = "Date",
ylab = "Predictive Signal")
# Create Psi-Plot
p3 <- plot(x = as.Date(oos_dates),
y = oos_chosen_psi,
ylim = c(1, 100),
main = "Evolution of the subset size",
type = "p",
cex = 0.75,
pch = 20,
xlab = "Date",
ylab = "Psi")
# Relative MSE
print(paste("Relative MSE:", round(mse[[1]] / mse[[2]], 4)))
# Print Plots
print(p1)
print(p2)
print(p3)
Authors
Philipp Adämmer, Sven Lehmann and Rainer Schüssler
License
GPL (>= 2)