Description
Linear Specification Testing.
Description
Tests whether the linear hypothesis of a model is correct specified using Dominguez-Lobato test. Also Ramsey's RESET (Regression Equation Specification Error Test) test is implemented and Wald tests can be carried out. Although RESET test is widely used to test the linear hypothesis of a model, Dominguez and Lobato (2019) proposed a novel approach that generalizes well known specification tests such as Ramsey's. This test relies on wild-bootstrap; this package implements this approach to be usable with any function that fits linear models and is compatible with the update() function such as 'stats'::lm(), 'lfe'::felm() and 'forecast'::Arima(), for ARMA (autoregressive–moving-average) models. Also the package can handle custom statistics such as Cramer von Mises and Kolmogorov Smirnov, described by the authors, and custom distributions such as Mammen (discrete and continuous) and Rademacher. Manuel A. Dominguez & Ignacio N. Lobato (2019) <doi:10.1080/07474938.2019.1687116>.
README.md
lineartestr
The goal of lineartestr
is to contrast the linear hypothesis of a model:
Using the Domínguez-Lobato test which relies on wild-bootstrap. Also the Ramsey RESET test is implemented.
Installation
You can install the released version of lineartestr
from CRAN with:
install.packages("lineartestr")
And the development version from GitHub with:
# install.packages("devtools")
devtools::install_github("FedericoGarza/lineartestr")
References
- Manuel A. Domínguez and Ignacio N. Lobato (2019). Specification testing with estimated variables. Econometric Reviews.
Cite as
- Garza F (2020). lineartestr: Test the linear specification of a model. R package version 1.0.0, https://github.com/FedericoGarza/lineartestr.
Examples
Simplest linear models using lm
function
library(lineartestr)
x <- 1:100
y <- 1:100
lm_model <- lm(y~x)
dl_test <- dominguez_lobato_test(lm_model)
dplyr::glimpse(dl_test$test)
#> Observations: 1
#> Variables: 7
#> $ name_distribution <chr> "rnorm"
#> $ name_statistic <chr> "cvm_value"
#> $ statistic <dbl> 7.562182e-29
#> $ p_value <dbl> 0.4066667
#> $ quantile_90 <dbl> 2.549223e-28
#> $ quantile_95 <dbl> 3.887169e-28
#> $ quantile_99 <dbl> 6.359681e-28
Also lineartestr
can plot the results
plot_dl_test(dl_test)
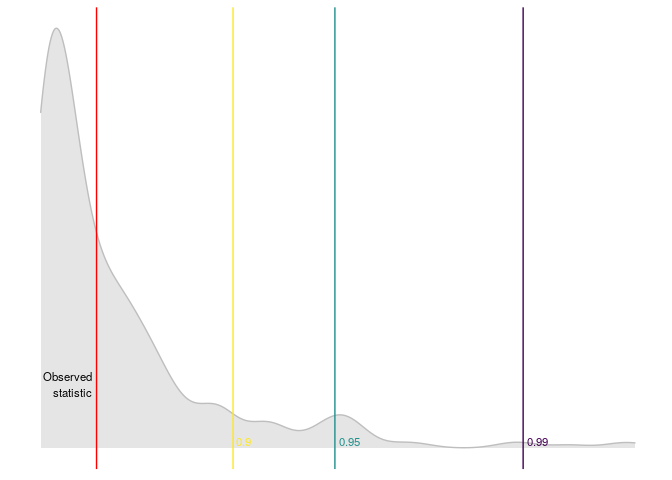
Run in parallel!
library(lineartestr)
x_p <- 1:1e5
y_p <- 1:1e5
lm_model_p <- lm(y_p~x_p)
dl_test_p <- dominguez_lobato_test(lm_model_p, n_cores=7)
dplyr::glimpse(dl_test_p$test)
#> Observations: 1
#> Variables: 7
#> $ name_distribution <chr> "rnorm"
#> $ name_statistic <chr> "cvm_value"
#> $ statistic <dbl> 6.324343e-21
#> $ p_value <dbl> 0.3566667
#> $ quantile_90 <dbl> 1.902697e-20
#> $ quantile_95 <dbl> 2.532663e-20
#> $ quantile_99 <dbl> 4.141126e-20
RESET test can also be used to test the linear hypothesis
library(lineartestr)
x <- 1:100 + rnorm(100)
y <- 1:100
lm_model <- lm(y~x)
r_test <- reset_test(lm_model)
dplyr::glimpse(r_test)
#> Observations: 1
#> Variables: 6
#> $ statistic <dbl> 0.7797557
#> $ p_value <dbl> 0.6771396
#> $ df <int> 2
#> $ quantile_90 <dbl> 4.60517
#> $ quantile_95 <dbl> 5.991465
#> $ quantile_99 <dbl> 9.21034
An then we can plot the results
plot_reset_test(r_test)
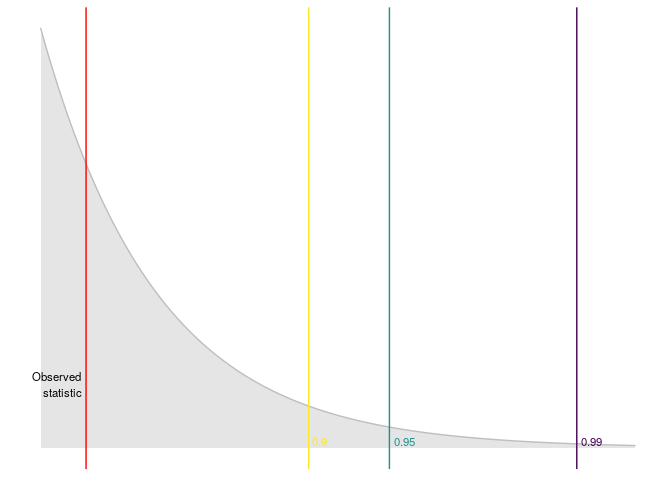
Linear fixed effects with lfe
library(lineartestr)
library(dplyr)
#>
#> Attaching package: 'dplyr'
#> The following objects are masked from 'package:stats':
#>
#> filter, lag
#> The following objects are masked from 'package:base':
#>
#> intersect, setdiff, setequal, union
library(lfe)
#> Loading required package: Matrix
# This example was taken from https://www.rdocumentation.org/packages/lfe/versions/2.8-5/topics/felm
x <- rnorm(1000)
x2 <- rnorm(length(x))
# Individuals and firms
id <- factor(sample(20,length(x),replace=TRUE))
firm <- factor(sample(13,length(x),replace=TRUE))
# Effects for them
id.eff <- rnorm(nlevels(id))
firm.eff <- rnorm(nlevels(firm))
# Left hand side
u <- rnorm(length(x))
y <- x + 0.5*x2 + id.eff[id] + firm.eff[firm] + u
new_y <- y + rnorm(length(y))
## Estimate the model
est <- lfe::felm(y ~ x + x2 | id + firm)
## Testing the linear hypothesis and plotting results
dominguez_lobato_test(est, n_cores = 7) %>%
plot_dl_test()
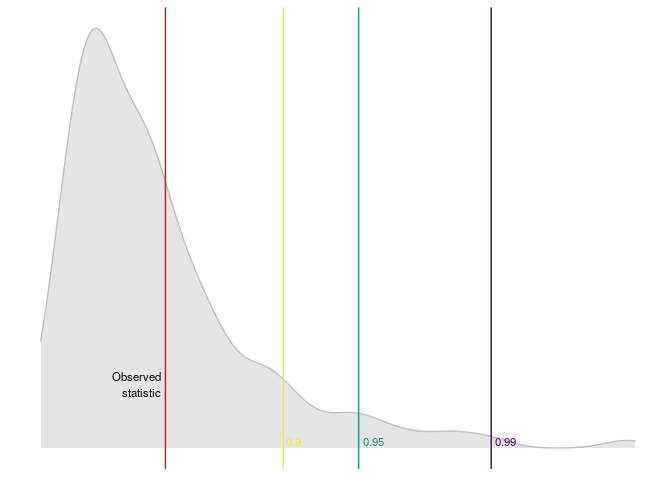
ARMA models
library(lineartestr)
library(dplyr)
x <- rnorm(100)**3
arma_model <- forecast::Arima(x, order = c(1, 0, 1))
#> Registered S3 method overwritten by 'xts':
#> method from
#> as.zoo.xts zoo
#> Registered S3 method overwritten by 'quantmod':
#> method from
#> as.zoo.data.frame zoo
#> Registered S3 methods overwritten by 'forecast':
#> method from
#> fitted.fracdiff fracdiff
#> residuals.fracdiff fracdiff
dominguez_lobato_test(arma_model) %>%
plot_dl_test()
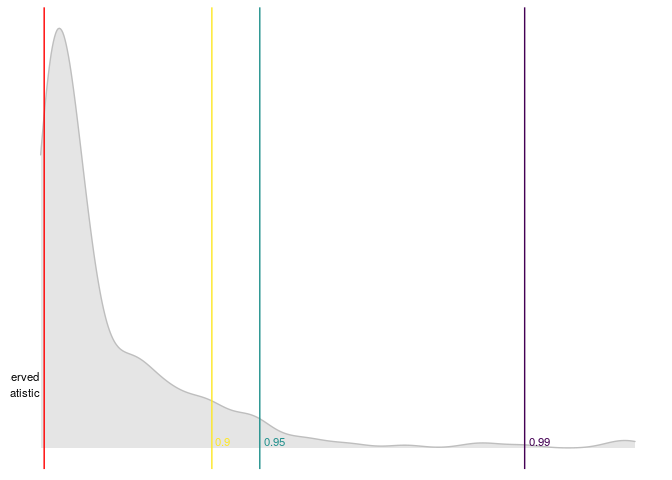