Analysis of Broth Microdilution Assays.
microdiluteR 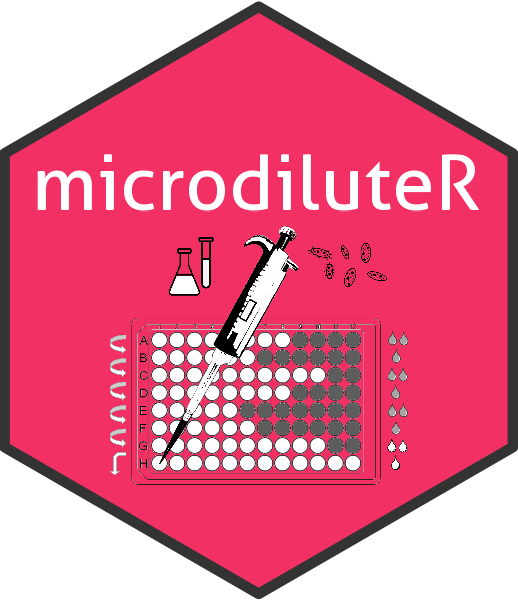
:notebook: Background
The microdiluteR
package is designed to help researchers tidy up data from photometer plates and provides functions to easily add metadata, regardless of whether the user is processing a single plate or multiple plates with complex metadata structures. This package was developed with a special focus on the analysis of broth microdilution assays. A detailed tutorial can be found here.
:floppy_disk: Installation
You can install the development version of microdiluteR
from GitHub with:
# install.packages("devtools") # if not installed already
devtools::install_github("silvia-eckert/microdiluteR")
:joystick: Usage
You can load microdiluteR
as follows:
library(microdiluteR)
Let’s try out the main function tidy_plates()
with example data:
data(bma)
bma[1] # file name is bma_grp1_exp2_T0
#> $bma_grp1_exp2_T0
#> 1 2 3 4 5 6 7 8 9 10 11 12
#> A 0.342 0.354 0.360 0.360 0.352 0.363 0.361 0.352 0.356 0.351 0.366 0.375
#> B 0.362 0.391 0.375 0.363 0.383 0.366 0.380 0.378 0.339 0.387 0.377 0.362
#> C 0.344 0.346 0.345 0.347 0.350 0.356 0.348 0.343 0.348 0.351 0.351 0.353
#> D 0.361 0.367 0.351 0.364 0.353 0.362 0.361 0.367 0.363 0.356 0.357 0.355
#> E 0.388 0.473 0.400 0.358 0.388 0.340 0.335 0.396 0.411 0.404 0.397 0.407
#> F 0.456 0.465 0.469 0.469 0.462 0.468 0.455 0.477 0.487 0.488 0.498 0.471
#> G 0.334 0.340 0.357 0.332 0.329 0.342 0.333 0.317 0.360 0.332 0.335 0.328
#> H 0.334 0.332 0.339 0.333 0.339 0.334 0.342 0.335 0.361 0.327 0.330 0.341
For the example data, the corresponding metadata is stored as an attribute:
attr(bma, "metadata")
#> plate_axis treatment concentration
#> 1 A 10% 100 ppm
#> 2 B 10% 200 ppm
#> 3 C 30% 100 ppm
#> 4 D 30% 200 ppm
#> 5 E 100% 100 ppm
#> 6 F 100% 200 ppm
#> 7 G Control 100 ppm
#> 8 H Control 200 ppm
Let’s add the metadata and create a tidy data frame for further processing:
tidy_data <- tidy_plates(bma[1],
how_many = "single",
direction = "horizontal",
validity_method = "threshold",
threshold = 0.355, # values above this are set as invalid
group_ID = "Group 1", # optional
experiment_name = "Experiment A", # optional
treatment_labels = rep(c("10%", "30%", "100%", "Control"), each = 2),
concentration_levels = rep(c(100,200), times = 4))
# Let's rename some columns for convenience
names(tidy_data)[names(tidy_data) == 'Position'] <- 'Pos'
names(tidy_data)[names(tidy_data) == 'Value'] <- 'Val'
names(tidy_data)[names(tidy_data) == 'Treatment'] <- 'Treat'
names(tidy_data)[names(tidy_data) == 'Concentration'] <- 'Conc'
names(tidy_data)[names(tidy_data) == 'Timepoint'] <- 'TP'
This is the resulting table:
tidy_data
#> # A tibble: 96 × 9
#> Pos Val Validity Treat Conc TP File Group Experiment
#> <chr> <dbl> <chr> <chr> <dbl> <chr> <chr> <chr> <chr>
#> 1 A-1 0.342 valid 10% 100 T0 bma_grp1_exp2_T0 Group 1 Experiment A
#> 2 A-2 0.354 valid 10% 100 T0 bma_grp1_exp2_T0 Group 1 Experiment A
#> 3 A-3 0.36 invalid 10% 100 T0 bma_grp1_exp2_T0 Group 1 Experiment A
#> 4 A-4 0.36 invalid 10% 100 T0 bma_grp1_exp2_T0 Group 1 Experiment A
#> 5 A-5 0.352 valid 10% 100 T0 bma_grp1_exp2_T0 Group 1 Experiment A
#> 6 A-6 0.363 invalid 10% 100 T0 bma_grp1_exp2_T0 Group 1 Experiment A
#> 7 A-7 0.361 invalid 10% 100 T0 bma_grp1_exp2_T0 Group 1 Experiment A
#> 8 A-8 0.352 valid 10% 100 T0 bma_grp1_exp2_T0 Group 1 Experiment A
#> 9 A-9 0.356 invalid 10% 100 T0 bma_grp1_exp2_T0 Group 1 Experiment A
#> 10 A-10 0.351 valid 10% 100 T0 bma_grp1_exp2_T0 Group 1 Experiment A
#> # ℹ 86 more rows
:heart: Logo generated with hexSticker.