Description
Cluster High Dimensional Categorical Datasets.
Description
Scalable Bayesian clustering of categorical datasets. The package implements a hierarchical Dirichlet (Process) mixture of multinomial distributions. It is thus a probabilistic latent class model (LCM) and can be used to reduce the dimensionality of hierarchical data and cluster individuals into latent classes. It can automatically infer an appropriate number of latent classes or find k classes, as defined by the user. The model is based on a paper by Dunson and Xing (2009) <doi:10.1198/jasa.2009.tm08439>, but implements a scalable variational inference algorithm so that it is applicable to large datasets. It is described and tested in the accompanying paper by Ahlmann-Eltze and Yau (2018) <doi:10.1109/DSAA.2018.00068>.
README.md
mixdir
The goal of mixdir is to cluster high dimensional categorical datasets.
It can
- handle missing data
- infer a reasonable number of latent class (try
mixdir(select_latent=TRUE)
) - cluster datasets with more than 70,000 observations and 60 features
- propagate uncertainty and produce a soft clustering
A detailed description of the algorithm and the features of the package can be found in the the accompanying paper. If you find the package useful please cite
C. Ahlmann-Eltze and C. Yau, "MixDir: Scalable Bayesian Clustering for High-Dimensional Categorical Data", 2018 IEEE 5th International Conference on Data Science and Advanced Analytics (DSAA), Turin, Italy, 2018, pp. 526-539.
Installation
install.packages("mixdir")
# Or to get the latest version from github
devtools::install_github("const-ae/mixdir")
Example
Clustering the mushroom data set.
# Loading the library and the data
library(mixdir)
set.seed(1)
data("mushroom")
# High dimensional dataset: 8124 mushroom and 23 different features
mushroom[1:10, 1:5]
#> bruises cap-color cap-shape cap-surface edible
#> 1 bruises brown convex smooth poisonous
#> 2 bruises yellow convex smooth edible
#> 3 bruises white bell smooth edible
#> 4 bruises white convex scaly poisonous
#> 5 no gray convex smooth edible
#> 6 bruises yellow convex scaly edible
#> 7 bruises white bell smooth edible
#> 8 bruises white bell scaly edible
#> 9 bruises white convex scaly poisonous
#> 10 bruises yellow bell smooth edible
Calling the clustering function mixdir
on a subset of the data:
# Clustering into 3 latent classes
result <- mixdir(mushroom[1:1000, 1:5], n_latent=3)
Analyzing the result
# Latent class of of first 10 mushrooms
head(result$pred_class, n=10)
#> [1] 3 1 1 3 2 1 1 1 3 1
# Soft Clustering for first 10 mushrooms
head(result$class_prob, n=10)
#> [,1] [,2] [,3]
#> [1,] 3.103495e-07 1.055098e-05 9.999891e-01
#> [2,] 9.998594e-01 4.683764e-06 1.359291e-04
#> [3,] 9.998944e-01 3.111462e-06 1.025194e-04
#> [4,] 5.778033e-04 7.114603e-08 9.994221e-01
#> [5,] 3.662625e-07 9.999992e-01 4.183025e-07
#> [6,] 9.996461e-01 8.764031e-08 3.537838e-04
#> [7,] 9.998944e-01 3.111462e-06 1.025194e-04
#> [8,] 9.997331e-01 5.822320e-08 2.668420e-04
#> [9,] 5.778033e-04 7.114603e-08 9.994221e-01
#> [10,] 9.999999e-01 5.850067e-09 9.845112e-08
pheatmap::pheatmap(result$class_prob, cluster_cols=FALSE,
labels_col = paste("Class", 1:3))
# Structure of latent class 1
# (bruises, cap color either yellow or white, edible etc.)
purrr::map(result$category_prob, 1)
#> $bruises
#> bruises no
#> 0.9998223256 0.0001776744
#>
#> $`cap-color`
#> brown gray red white yellow
#> 0.0001775934 0.0001819672 0.0001776373 0.4079822666 0.5914805356
#>
#> $`cap-shape`
#> bell convex flat sunken
#> 0.3926736 0.4767291 0.1304197 0.0001776
#>
#> $`cap-surface`
#> fibrous scaly smooth
#> 0.0568571 0.4871396 0.4560033
#>
#> $edible
#> edible poisonous
#> 0.9998223174 0.0001776826
# The most predicitive features for each class
find_predictive_features(result, top_n=3)
#> column answer class probability
#> 19 cap-color yellow 1 0.9993990
#> 22 cap-shape bell 1 0.9990947
#> 1 bruises bruises 1 0.7089533
#> 48 edible poisonous 3 0.9980468
#> 15 cap-color red 3 0.8462032
#> 9 cap-color brown 3 0.6473043
#> 5 bruises no 2 0.9990364
#> 11 cap-color gray 2 0.9978218
#> 32 cap-shape sunken 2 0.9936162
# For example: if all I know about a mushroom is that it has a
# yellow cap, then I am 99% certain that it will be in class 1
predict(result, c(`cap-color`="yellow"))
#> [,1] [,2] [,3]
#> [1,] 0.999399 0.0003004692 0.0003004907
# Note the most predictive features are different from the most typical ones
find_typical_features(result, top_n=3)
#> column answer class probability
#> 1 bruises bruises 1 0.9998223
#> 43 edible edible 1 0.9998223
#> 19 cap-color yellow 1 0.5914805
#> 3 bruises bruises 3 0.9995546
#> 27 cap-shape convex 3 0.7460615
#> 9 cap-color brown 3 0.6746224
#> 44 edible edible 2 0.9995310
#> 5 bruises no 2 0.9713177
#> 35 cap-surface fibrous 2 0.7355413
Dimensionality Reduction
# Defining Features
def_feat <- find_defining_features(result, mushroom[1:1000, 1:5], n_features = 3)
print(def_feat)
#> $features
#> [1] "cap-color" "bruises" "edible"
#>
#> $quality
#> [1] 74.35146
# Plotting the most important features gives an immediate impression
# how the cluster differ
plot_features(def_feat$features, result$category_prob)
#> Loading required namespace: ggplot2
#> Loading required namespace: tidyr
Underlying Model
The package implements a variational inference algorithm to solve a Bayesian latent class model (LCM).
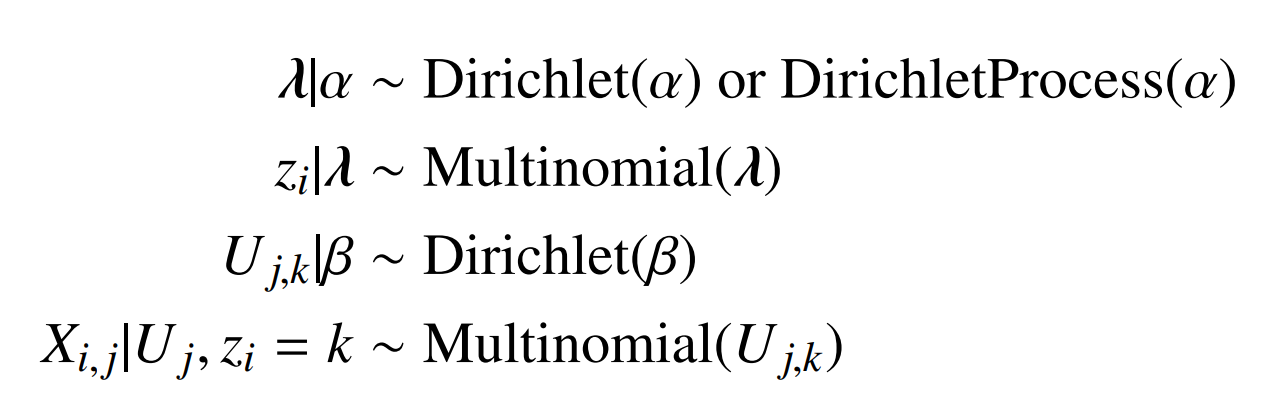