Interface to the Penn Machine Learning Benchmarks Data Repository.
pmlbr 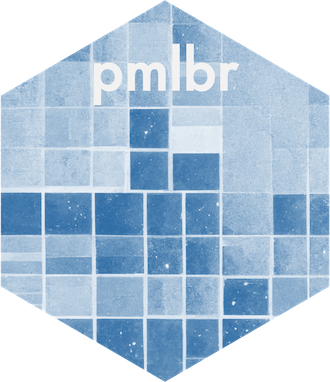
pmlbr is an R interface to the Penn Machine Learning Benchmarks (PMLB) data repository, a large collection of curated benchmark datasets for evaluating and comparing supervised machine learning algorithms. These datasets cover a broad range of applications including binary/multi-class classification and regression problems as well as combinations of categorical, ordinal, and continuous features.
This repository is originally forked from makeyourownmaker/pmlblite. We thank the pmlblite’s author for releasing the source code under the GPL-2 license so that others could reuse the software.
Install
This package works for any recent version of R.
You can install the released version of pmlbr from CRAN with:
install.packages("pmlbr")
Or the development version from GitHub with remotes:
# install.packages('remotes') # uncomment to install remotes
library(remotes)
remotes::install_github("EpistasisLab/pmlbr")
Usage
The core function of this package is fetch_data
that allows us to download data from the PMLB repository. For example:
library(pmlbr)
# Download features and labels for penguins dataset in single data frame
penguins <- fetch_data("penguins")
str(penguins)
## 'data.frame': 333 obs. of 8 variables:
## $ island : int 2 2 2 2 2 2 2 2 2 2 ...
## $ bill_length_mm : num 39.1 39.5 40.3 36.7 39.3 38.9 39.2 41.1 38.6 34.6 ...
## $ bill_depth_mm : num 18.7 17.4 18 19.3 20.6 17.8 19.6 17.6 21.2 21.1 ...
## $ flipper_length_mm: int 181 186 195 193 190 181 195 182 191 198 ...
## $ body_mass_g : int 3750 3800 3250 3450 3650 3625 4675 3200 3800 4400 ...
## $ sex : int 1 0 0 0 1 0 1 0 1 1 ...
## $ year : int 2007 2007 2007 2007 2007 2007 2007 2007 2007 2007 ...
## $ target : int 0 0 0 0 0 0 0 0 0 0 ...
## - attr(*, "na.action")= 'omit' Named int [1:11] 4 9 10 11 12 48 179 219 257 269 ...
## ..- attr(*, "names")= chr [1:11] "4" "9" "10" "11" ...
# Download features and labels for penguins dataset in separate data structures
penguins <- fetch_data("penguins", return_X_y = TRUE)
head(penguins$x) # data frame
## island bill_length_mm bill_depth_mm flipper_length_mm body_mass_g sex year
## 1 2 39.1 18.7 181 3750 1 2007
## 2 2 39.5 17.4 186 3800 0 2007
## 3 2 40.3 18.0 195 3250 0 2007
## 4 2 NA NA NA NA NA 2007
## 5 2 36.7 19.3 193 3450 0 2007
## 6 2 39.3 20.6 190 3650 1 2007
head(penguins$y) # vector
## [1] 0 0 0 0 0 0
Let’s check other available datasets and their summary statistics:
# Dataset names
head(classification_dataset_names, 9)
## [1] "adult" "agaricus_lepiota" "allbp"
## [4] "allhyper" "allhypo" "allrep"
## [7] "analcatdata_aids" "analcatdata_asbestos" "analcatdata_authorship"
head(regression_dataset_names, 9)
## [1] "1027_ESL" "1028_SWD" "1029_LEV"
## [4] "1030_ERA" "1089_USCrime" "1096_FacultySalaries"
## [7] "1191_BNG_pbc" "1193_BNG_lowbwt" "1196_BNG_pharynx"
# Dataset summaries
head(summary_stats)
## dataset n_instances n_features n_binary_features
## 1 1027_ESL 488 4 0
## 2 1028_SWD 1000 10 0
## 3 1029_LEV 1000 4 0
## 4 1030_ERA 1000 4 0
## 5 1089_USCrime 47 13 0
## 6 1096_FacultySalaries 50 4 0
## n_categorical_features n_continuous_features endpoint_type n_classes
## 1 0 4 continuous 9
## 2 0 10 continuous 4
## 3 0 4 continuous 5
## 4 0 4 continuous 9
## 5 0 13 continuous 42
## 6 0 4 continuous 39
## imbalance task
## 1 0.099363200 regression
## 2 0.108290667 regression
## 3 0.111245000 regression
## 4 0.031251250 regression
## 5 0.002970111 regression
## 6 0.004063158 regression
Selecting a subset of datasets that satisfy certain conditions is straight forward with dplyr
. For example, if we need datasets with fewer than 100 observations for a classification task:
library(dplyr)
summary_stats %>%
filter(n_instances < 100, task == "classification") %>%
pull(dataset)
## [1] "analcatdata_aids" "analcatdata_asbestos"
## [3] "analcatdata_bankruptcy" "analcatdata_cyyoung8092"
## [5] "analcatdata_cyyoung9302" "analcatdata_fraud"
## [7] "analcatdata_happiness" "analcatdata_japansolvent"
## [9] "confidence" "labor"
## [11] "lupus" "parity5"
## [13] "postoperative_patient_data"
Dataset format
All data sets are stored in a common format:
- First row is the column names
- Each following row corresponds to an individual observation
- The target column is named
target
- All columns are tab (
\t
) separated - All files are compressed with
gzip
to conserve space
This R library includes summaries of the classification and regression data sets but does not store any of the PMLB data sets. The data sets can be downloaded using the fetch_data
function which is similar to the corresponding PMLB python function.
Further info:
?fetch_data
?summary_stats
Citing
If you use PMLB in a scientific publication, please consider citing one of the following papers:
Joseph D. Romano, Le, Trang T., William La Cava, John T. Gregg, Daniel J. Goldberg, Praneel Chakraborty, Natasha L. Ray, Daniel Himmelstein, Weixuan Fu, and Jason H. Moore. PMLB v1.0: an open source dataset collection for benchmarking machine learning methods.arXiv preprint arXiv:2012.00058 (2020).
Randal S. Olson, William La Cava, Patryk Orzechowski, Ryan J. Urbanowicz, and Jason H. Moore (2017). PMLB: a large benchmark suite for machine learning evaluation and comparison. BioData Mining 10, page 36.
Roadmap
- Add tests
Contributing
Pull requests are welcome. For major changes, please open an issue first to discuss what you would like to change.
Integration of other data repositories are particularly welcome.
Alternatives
- Penn Machine Learning Benchmarks
- OpenML Approximately 2,500 datasets - available for download using R module
- UC Irvine Machine Learning Repository
- mlbench: Machine Learning Benchmark Problems
- Rdatasets: An archive of datasets distributed with R
- datasets.load: Visual interface for loading datasets in RStudio from all installed (unloaded) packages
- stackoverflow: How do I get a list of built-in data sets in R?