Penalized Semiparametric Bayesian Cox Models.
psbcSpeedUp
This is a C++ speed-up and extended version of the R-pakcage psbcGroup. It implements the Bayesian Lasso Cox model (Lee et al., 2011) and the Bayesian Lasso Cox with mandatory variables (Zucknick et al., 2015). Bayesian Lasso Cox models with other shrinkage and group priors (Lee et al., 2015) are to be implemented later on.
Installation
Install the latest released version from CRAN
install.packages("psbcSpeedUp")
Install the latest development version from GitHub
#install.packages("remotes")
remotes::install_github("ocbe-uio/psbcSpeedUp")
Examples
Run a Bayesian Lasso Cox with mandatory variables
Data set exampleData
consists of six components: survival times t
, event status di
, covariates x
, number of genomics variables p
, number of clinical variables q
and true effects of covariates beta_true
. See ?exampleData
for more information of the data.
To run a Bayesian Lasso Cox model for variable selection of the first $p$ genomics variables and inclusion of $q$ mandatory variables, one can specify arguments of the main function psbcSpeedUp()
with p = p
and q = q
. If the arguments p
and q
are unspecified, the Bayesian Lasso Cox model does variable selection for all covariates, i.e., by default p = ncol(survObj$x)
and q = 0
.
# Load the example dataset
data("exampleData", package = "psbcSpeedUp")
p <- exampleData$p
q <- exampleData$q
survObj <- exampleData[1:3]
# Set hyperparameters (see help file for specifying more hyperparameters)
mypriorPara <- list('eta0'=0.02, 'kappa0'=1, 'c0'=2, 'r'=10/9, 'delta'=1e-05,
'lambdaSq'=1, 'sigmaSq'= runif(1, 0.1, 10), 'beta.prop.var'=1, 'beta.clin.var'=1)
# run Bayesian Lasso Cox
library("psbcSpeedUp")
set.seed(123)
fitBayesCox <- psbcSpeedUp(survObj, p=p, q=q, hyperpar=mypriorPara,
nIter=1000, burnin=500, outFilePath="/tmp")
Running MCMC iterations ...
[##################################################] 100%
DONE, exiting!
Plot posterior estimates of regression cofficients
The function psbcSpeedUp::plot()
can show the posterior mean and 95% credible intervals of regression coefficients.
plot(fitBayesCox)
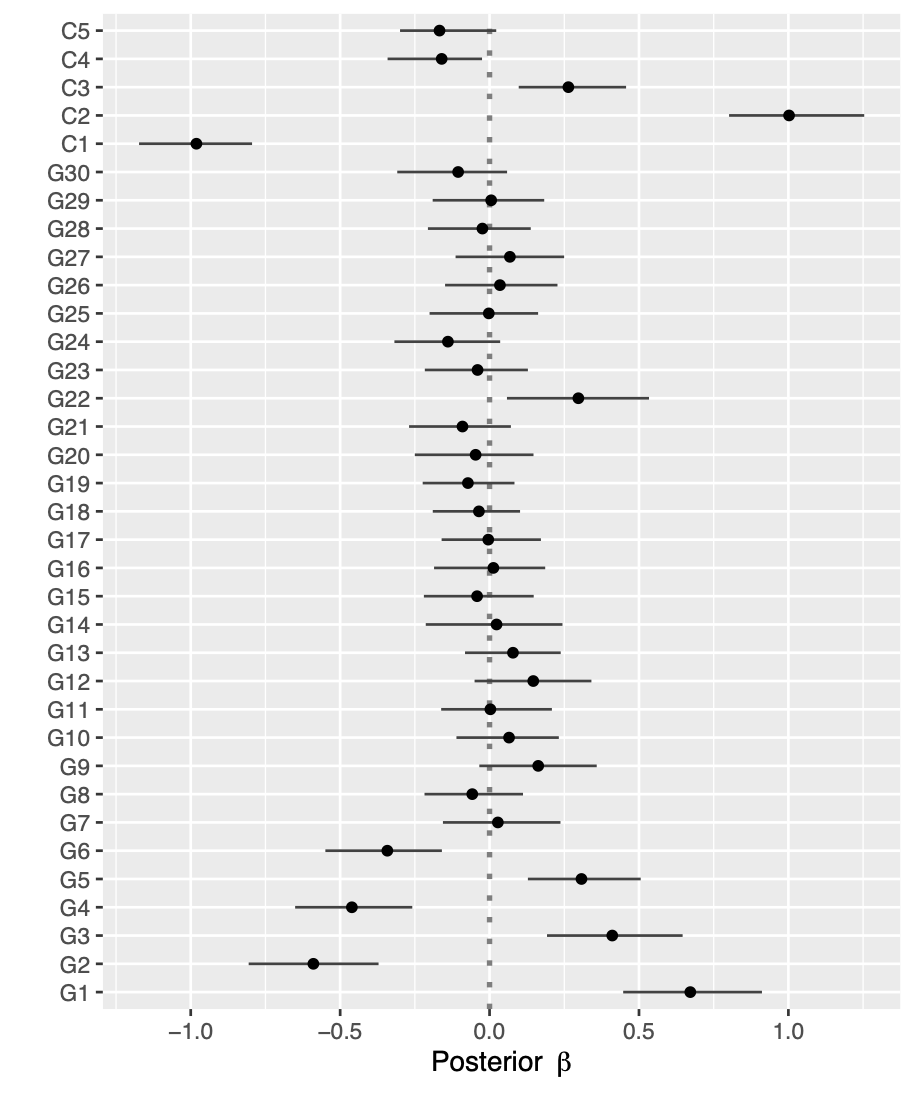
Plot time-dependent Brier scores
The function psbcSpeedUp::plotBrier()
can show the time-dependent Brier scores based on posterior mean of coefficients or Bayesian model averaging.
plotBrier(fitBayesCox, times = 80)
Null.model Bayesian.Cox
IBS 0.2089742 0.109274
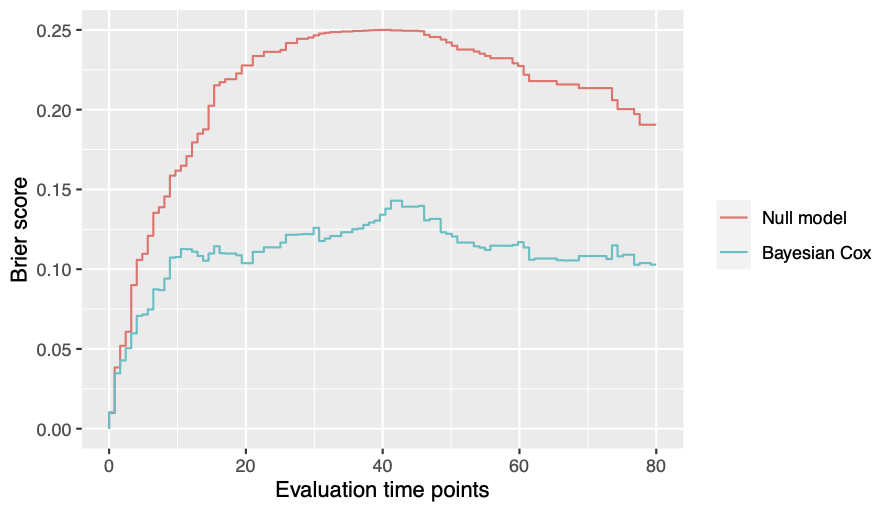
Predict survival probabilities and cumulative hazards
The function psbcSpeedUp::predict()
can estimate the survival probabilities and cumulative hazards.
predict(fitBayesCox, type = c("cumhazard", "survival"))
## observation times cumhazard survival
## 1: 1 0.264 1.08e-05 1.00e+00
## 2: 2 0.264 4.50e-05 1.00e+00
## 3: 3 0.264 5.33e-05 1.00e+00
## 4: 4 0.264 1.84e-05 1.00e+00
## 5: 5 0.264 7.22e-05 1.00e+00
## ---
## 39996: 196 107.641 2.66e+00 6.97e-02
## 39997: 197 107.641 5.47e-01 5.79e-01
## 39998: 198 107.641 5.15e+01 4.41e-23
## 39999: 199 107.641 4.13e+02 5.72e-180
## 40000: 200 107.641 2.24e-01 7.99e-01
References
Kyu Ha Lee, Sounak Chakraborty, Jianguo Sun (2011). Bayesian variable selection in semiparametric proportional hazards model for high dimensional survival data. The International Journal of Biostatistics, 7:1. DOI: 10.2202/1557-4679.1301.
Kyu Ha Lee, Sounak Chakraborty, Jianguo Sun (2015). Survival prediction and variable selection with simultaneous shrinkage and grouping priors. Statistical Analysis and Data Mining, 8:114-127. DOI:10.1002/sam.11266.
Manuela Zucknick, Maral Saadati, Axel Benner (2015). Nonidentical twins: Comparison of frequentist and Bayesian lasso for Cox models. Biometrical Journal, 57:959-981. DOI:10.1002/bimj.201400160.