Description
Tools for Data Splitting.
Description
Fast, lightweight toolkit for data splitting. Data sets can be partitioned into disjoint groups (e.g. into training, validation, and test) or into (repeated) k-folds for subsequent cross-validation. Besides basic splits, the package supports stratified, grouped as well as blocked splitting. Furthermore, cross-validation folds for time series data can be created. See e.g. Hastie et al. (2001) <doi:10.1007/978-0-387-84858-7> for the basic background on data partitioning and cross-validation.
README.md
{splitTools} 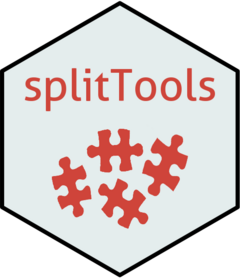
Overview
{splitTools} is a toolkit for fast data splitting. It does not have any dependencies.
Its two main functions partition()
and create_folds()
support
- data partitioning (e.g. into training, validation and test),
- creating (in- or out-of-sample) folds for cross-validation (CV),
- creating repeated folds for CV,
- stratified splitting,
- grouped splitting as well as
- blocked splitting (if the sequential order of the data should be retained).
The function create_timefolds()
does time-series splitting where the out-of-sample data follows the (extending or moving) in-sample data.
The result of create_folds()
can be directly passed to the folds
argument in CV functions of XGBoost or LightGBM. Since these functions expect out-of-sample indices, set the option invert = TRUE
.
Installation
# From CRAN
install.packages("splitTools")
# Development version
devtools::install_github("mayer79/splitTools")
Usage
library(splitTools)
p <- c(train = 0.5, valid = 0.25, test = 0.25)
# Train/valid/test indices for iris data stratified by Species
str(inds <- partition(iris$Species, p, seed = 1))
# List of 3
# $ train: int [1:73] 1 3 5 7 8 10 12 13 14 15 ...
# $ valid: int [1:38] 4 9 19 21 27 28 29 30 32 35 ...
# $ test : int [1:39] 2 6 11 16 18 22 26 37 38 40 ...
# Same, but different output interface
head(inds <- partition(iris$Species, p, split_into_list = FALSE, seed = 1))
# [1] train test train valid train test
# Levels: train valid test
# In-sample indices for 5-fold CV (stratified by Species)
str(inds <- create_folds(iris$Species, k = 5, seed = 1))
# List of 5
# $ Fold1: int [1:120] 2 4 5 6 7 8 9 10 11 15 ...
# $ Fold2: int [1:120] 1 2 3 4 5 6 9 10 11 12 ...
# $ Fold3: int [1:120] 1 2 3 4 6 7 8 9 11 12 ...
# $ Fold4: int [1:120] 1 3 5 6 7 8 10 11 12 13 ...
# $ Fold5: int [1:120] 1 2 3 4 5 7 8 9 10 12 ...
# In-sample indices for 3 times repeated 5-fold CV (stratified by Species)
str(inds <- create_folds(iris$Species, k = 5, m_rep = 3, seed = 1))
# List of 15
# $ Fold1.Rep1: int [1:120] 2 4 5 6 7 8 9 10 11 15 ...
# $ Fold2.Rep1: int [1:120] 1 2 3 4 5 6 9 10 11 12 ...
# $ Fold3.Rep1: int [1:120] 1 2 3 4 6 7 8 9 11 12 ...
# $ Fold4.Rep1: int [1:120] 1 3 5 6 7 8 10 11 12 13 ...
# $ Fold5.Rep1: int [1:120] 1 2 3 4 5 7 8 9 10 12 ...
# $ Fold1.Rep2: int [1:120] 1 2 3 4 5 6 8 9 11 12 ...
# $ Fold2.Rep2: int [1:120] 1 3 6 7 8 9 10 12 13 14 ...
# [...]
# Indices for time-series splitting
str(inds <- create_timefolds(1:100, k = 5))
# List of 5
# $ Fold1:List of 2
# ..$ insample : int [1:17] 1 2 3 4 5 6 7 8 9 10 ...
# ..$ outsample: int [1:17] 18 19 20 21 22 23 24 25 26 27 ...
# $ Fold2:List of 2
# ..$ insample : int [1:34] 1 2 3 4 5 6 7 8 9 10 ...
# ..$ outsample: int [1:17] 35 36 37 38 39 40 41 42 43 44 ...
# $ Fold3:List of 2
# [...]
For more details, check out the vignette.